Recruitment in the Mental Health Treatment Study: A Behavioral Health/Employment Intervention for Social Security Disabled-Worker Beneficiaries
Social Security Bulletin, Vol. 74, No. 2, 2014
This article analyzes subject recruitment for the Mental Health Treatment Study (MHTS)—a national 23-site randomized trial that provided access to effective treatment and rehabilitation interventions for Social Security Disability Insurance (DI) beneficiaries with psychiatric impairments. We use regression analyses to better understand the likely take-up rate for MHTS replications and/or expansions and to identify characteristics of DI beneficiaries most likely to enroll. Results indicate that among potential MHTS subjects with confirmed telephone contacts, the take-up rate was 14.0 percent—well above rates for previous Social Security Administration randomized trials. Regression results suggest, as an upper bound, that take-up rates in the 18.0–25.0 percent range could be obtained by targeting recruitment to the group of beneficiaries that has administrative records of recent vocational or labor-market activity. Future interventions with large, heterogeneous target populations should consider the implications here for generalizing intervention impacts and modifying recruitment strategies.
David Salkever is a professor in the Department of Public Policy, University of Maryland Baltimore County (UMBC). Brent Gibbons is a post-doctoral fellow in the Maryland Institute for Policy Analysis and Research, UMBC. William D. Frey is vice president of Westat. Roline Milfort and Julie Bollmer are research associates with Westat. Thomas Hale is an economist in the Office of Retirement and Disability Policy, Social Security Administration. Robert Drake is a professor of psychiatry in the Geisel School of Medicine, Dartmouth College. Howard Goldman is a professor of psychiatry in the University of Maryland School of Medicine.
Acknowledgments: We thank Jarnee Riley and Sigurd Hermansen of Westat for providing valuable ongoing assistance in understanding and interpreting the various data sources and items that we used during the preparation of this work. We also thank the anonymous referees for useful suggestions on the original version of this article.
This article extends work conducted under contract no. SS00-05-60072 between the Social Security Administration (SSA) and Westat; the analysis was conducted for the benefit of SSA.
The findings and conclusions presented in the Bulletin are those of the authors and do not necessarily represent the views of the Social Security Administration or any other author affiliations.
Introduction
DI | Disability Insurance |
IPS | individual placement and support |
MBR | Master Beneficiary Record |
MHTS | Mental Health Treatment Study |
RIG | research information group |
SE | supported employment |
SPMD | severe and persistent mental disorder |
SSA | Social Security Administration |
SSI | Supplemental Security Income |
TTW | Ticket to Work |
TWP | trial work period |
Although promoting the return to work of Social Security Disability Insurance (DI) beneficiaries has long been an objective of the Social Security Administration's (SSA's) policy initiatives, several factors argue for a special focus on beneficiaries with psychiatric impairments. Those beneficiaries are a large proportion of all DI beneficiaries; in 2011, they accounted for 29.1 percent of all Social Security disabled-worker beneficiaries younger than age 50.1 Moreover, disabled workers with psychiatric impairments tend to go on the DI rolls at younger ages than other beneficiaries.
Access to effective behavioral health treatment and vocational rehabilitation for DI beneficiaries with psychiatric impairments is also a relevant concern for SSA and other federal agencies. A number of behavioral health treatment and rehabilitation interventions for persons with severe and persistent mental disorders (SPMDs) have demonstrated evidence-based effectiveness.2 However, Medicare coverage for these interventions (as well as Medicaid coverage for individuals receiving concurrent Supplemental Security Income (SSI) payments and DI benefits) has important gaps. Thus, large numbers of beneficiaries with SPMDs do not benefit from these effective interventions, and, as a result, do not achieve attainable improvements in their functioning.3
In response to available intervention concerns, SSA implemented the Mental Health Treatment Study (MHTS)—a national randomized trial that provided access to effective treatment and rehabilitation interventions for DI beneficiaries with psychiatric impairments. Those beneficiaries randomized to the MHTS treatment group received (1) supported employment (SE) services following the evidence-based individual placement and support (IPS) template, (2) systematic medication management services to monitor and manage their pharmacological treatments, (3) enhanced insurance coverage for behavioral health care, and (4) reimbursement of out-of-pocket behavioral health or work-related expenses (transportation, copays, and so forth). In addition, SSA's requirement for continuing disability reviews was waived for a 3-year period for the treatment group.
Item 1, IPS-SE services, was directed specifically at helping beneficiaries return to work and was thus provided to all persons in the treatment group. Those services were provided by a local supported employment agency in each of the 23 study sites. The IPS template for SE services includes the following critical elements:
- Consumer choice through the provision of services to all consumers interested in employment.
- Integration of vocational services with the overall mental health treatment program.
- Placement in regular competitive job settings, with no mandatory requirements for preemployment training or prior placement in segregated/sheltered work.
- Rapid job search, with an employment specialist and the client beginning job searches shortly after enrollment in IPS.
- On-going follow-up services by the employment specialist, once the client is placed, for as long as services are needed.
- Service priorities and content based primarily on consumer preferences.
- Benefits counseling for all consumers.
Items 2, 3, and 4 were provided to treatment group beneficiaries on an as needed basis; IPS-SE services were provided to all persons in the treatment group.4
MHTS control group beneficiaries received a comprehensive manual of available community resources and services and a total payment of $100 for completing a baseline interview and eight follow-up quarterly interviews. These beneficiaries' access to and use of treatment and rehabilitation services were presumed to reflect “treatment as usual.”
Because access to evidence-based IPS-SE services was a critical component of the MHTS intervention, recruitment of beneficiaries to the trial was restricted to the geographic catchment areas of 23 sites around the country where agencies providing IPS-SE services were already in existence. Those sites were selected to provide geographic and demographic diversity; at least one site was located in each of the four census regions of the country, but there was a preponderance of sites in the eastern and midwestern states because of the concentration of agencies providing IPS-SE services in those areas of the country.
The MHTS trial was undertaken to assess the potential effectiveness of a program that makes this intervention package available on a national basis to DI beneficiaries with SPMDs. An important step in this assessment was to examine the MHTS recruitment experience to better understand the likely take-up rate for such a program and the characteristics of the DI beneficiaries most likely to enroll. Because the potential target population for such a program is large and heterogeneous, understanding the differences between study participants and nonparticipants is important for generalizing any results about intervention impacts to other groups of beneficiaries. Moreover, maximizing the cost effectiveness of actual future implementations will depend on efficient targeting of recruitment efforts to those groups of beneficiaries expected to have the highest take-up rates and to show the highest average benefit from the program.
The overall analysis in this article addresses the enrollment component of the MHTS. We begin with a brief description of the MHTS recruitment and enrollment procedures and follow with an overview of our analytic approach. We then discuss data sources and variables, followed by the results of our statistical analyses. The study results and implications for future policy and research, in addition to our conclusion, are taken up in the Discussion section.
Recruitment and Enrollment Processes in the MHTS
This section provides a brief description of our recruitment and enrollment procedures. We discuss the target population, the sampling process and selection of beneficiaries, and the recruitment process and its outcomes.
Target Population
The MHTS recruited DI beneficiaries who were (1) aged 18 to 55, (2) adjudicated as a disabled worker based on a diagnosis of schizophrenia or a mood disorder, and (3) within the primary or backup catchment areas of one of the selected study sites. The catchment areas for each site were determined via in-person meetings of MHTS staff with each of the SE provider agencies. The areas were defined based on five-digit zip codes, with consideration of distances from the sites as well as political jurisdiction boundaries. Primary catchment areas generally included formally specified service area boundaries for sites (when they existed) and/or zip codes within a radius of about 30 miles from each site. Back-up areas included persons residing near to but outside of the primary catchment areas.
SSA provided study investigators with a data file on 61,530 DI beneficiaries from the Master Beneficiary Record (MBR) files who met the three primary inclusion criteria. Application of additional exclusion criteria based on MBR data—for persons with a legal guardian and/or residing in a nursing home or custodial institution—eliminated 1,499 of those beneficiaries from the target population.
Sampling Process and Selection of Beneficiaries to Contact
Names and contact information on beneficiaries eligible for the study were released (in blocks of 25 persons at a time) to the study sites for recruitment efforts. Random sampling was used to select beneficiaries for release, with the initial samples for each site coming from their primary catchment areas. The target enrollment was initially set at 3,000 persons distributed evenly across all sites.
The process of recruiting the selected beneficiaries began in October 2006. Initially, beneficiaries not enrolled in SSI but who were on the DI rolls for less than 24 months were excluded because of concerns about their lack of health insurance coverage and possible expense to the MHTS for covering them for behavioral health services. However, near the end of the first 12 months of recruitment, that group was subsequently sampled at the same rate as other beneficiaries (so their underrepresentation was limited to those initial months of the recruiting process.)
Because recruitment proceeded at a slower pace than originally projected, beneficiaries from the back-up catchment areas were released to the sites for recruitment, and the overall target was reduced from 3,000 to 2,200 persons. July 31, 2008, was set as the date for terminating recruitment outreach for all sites; with the completion of recruitment activities already in process by that date, the final number of enrollees was 2,238.
Recruitment Process
The process for recruiting each beneficiary involved five steps. First, a letter was printed and mailed—along with a brochure about the study and a return response card—to the contact name and address of the beneficiary as provided by SSA. The letter explained the study and invited the beneficiary to attend a research information group (RIG) meeting. Second, 3 to 5 days after mailing the letter, the research assistant (RA) at each site made one or more follow-up telephone calls to the beneficiary to confirm receipt of the introductory mailing, update or add to the beneficiary's contact information, provide further explanation of the study if needed, invite the beneficiary to attend an RIG meeting, and to confirm that the beneficiary met the three primary inclusion criteria.
Third, beneficiaries who were interested in enrollment attended in-person RIG meetings where RAs explained additional eligibility criteria, study randomization and procedures for treatment and control groups, and the content and schedule of study interviews. (The additional eligibility criteria were (1) no life-threatening physical conditions that would prevent study completion, (2) no competitive job within the month prior to study enrollment, and (3) no receipt of SE services from the study site within the 6 months prior to enrollment.) Interested beneficiaries were usually required to attend two RIG meetings, though some of them requested and received individual meetings with the same content. All beneficiaries who enrolled were required to attend at least two RIG or individual meetings on separate days to ensure their understanding of and commitment to the study.
Fourth, beneficiaries who wished to proceed with enrollment completed a brief in-person competency and health-screening interview conducted by the RA. This was used to verify that beneficiaries were competent to give consent and understood the nature of the study, to ensure that beneficiaries did not report a life-threatening physical condition, and to obtain signed enrollment consent forms. Fifth, beneficiaries completed a baseline interview and were then randomized to one of the two study groups (treatment or control).
As the foregoing description suggests, the recruitment process for each beneficiary involved multiple steps (including numerous follow-up contacts after the initial mailing of recruitment letters) and considerable time and effort on the part of RAs at the study sites. Given the demands on the RAs' time and a slower-than-projected rate of enrollment, Westat study staff began in November 2007 to assist the RAs at the sites by mailing initial recruitment letters and by making some of the initial follow-up calls. Finally, RAs were expected to document in Westat's Survey Management System every telephone contact or attempted contact with the beneficiaries being recruited; however, given the demands on their time, it is not surprising that this documentation process was incomplete.
Recruitment Outcomes
The flowchart in this article describes the outcomes of the recruitment process. Of the 61,530 beneficiaries in our target population, 31,785 were identified as not potential enrollees for one of the following reasons:
- 5,274 did not meet all study inclusion criteria;
- 14,397 could not be located;
- 4,166 were not mailed the initial recruitment letter; and
- 7,948 never received a follow-up call.
Some beneficiaries in the last two groups were not actively recruited because their sites ended recruitment efforts before letters could be printed and/or mailed or before follow-up calls could be made.
Of the remaining beneficiaries, 15,982 were identified as potential enrollees if (1) there was a study record of an RA telephone contact with that beneficiary and (2) the beneficiary met study eligibility requirements. Of those 15,982 potential enrollees, there were 3,971 who attended at least one RIG meeting (group or one-on-one), and of that group of beneficiaries, 2,238 were ultimately enrolled in the study. Finally, for an additional 13,763 beneficiaries who did not enroll, no records showed that a study RA had spoken with them. Because we could not ascertain whether those beneficiaries were potential enrollees who were never made aware of the study (and therefore could not possibly have enrolled), we classified them as possibly potential enrollees.
In this article, we examined the determinants of enrollment for both the 15,982 potential enrollees and for the combined set of 29,745 beneficiaries we classified as either potential enrollees or possibly potential enrollees. Thus, when focusing only on the potential enrollees, we observed a successful recruitment rate of 14.0 percent (2,238 out of 15,982); when including the possibly potential enrollees, that rate dropped to 7.5 percent.
Outcomes of the MHTS recruitment process
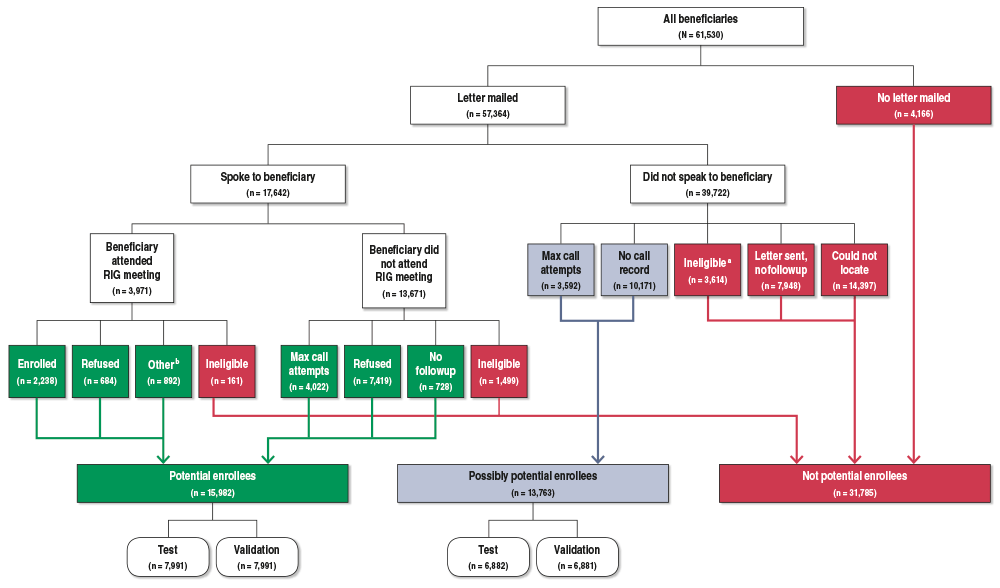
Text description for the flowchart.
Outcomes of the MHTS recruitment process
- All beneficiaries (N = 61,530).
- Letter mailed (n = 57,364).
- Spoke to beneficiary (n = 17,642).
- Beneficiary attended RIG meeting (n = 3,971).
- Enrolled (n = 2,238); thus, potential enrollees.
- Refused (n = 684); thus, potential enrollees.
- Other (n = 892); thus, potential enrollees. Includes beneficiaries who fell into one of three result codes: No longer locatable (n = 74), Max calls (n = 731), and Unknown (n = 87).
- Ineligible (n = 161); thus, not potential enrollees.
- Beneficiary did not attend RIG meeting (n = 13,671).
- Max call attempts (n = 4,022); thus, potential enrollees.
- Refused (n = 7,419); thus, potential enrollees.
- No followup (n = 728); thus, potential enrollees.
- Ineligible (n = 1,499); thus, not potential enrollees.
- Beneficiary attended RIG meeting (n = 3,971).
- Did not speak to beneficiary (n = 39,722).
- Max call attempts (n = 3,592); thus, possibly potential enrollees.
- No call record (n = 10,171); thus, possibly potential enrollees.
- Ineligible (n = 3,614); thus, not potential enrollees. Deemed ineligible for various reasons based on study-site records: The beneficiary was receiving supported employment services at the site, a proxy answered the recruitment call and indicated that the beneficiary had a legal guardian, and so forth.
- Letter sent, no followup (n = 7,948); thus, not potential enrollees.
- Could not locate (n = 14,397); thus, not potential enrollees.
- Spoke to beneficiary (n = 17,642).
- No letter mailed (n = 4,166); thus, not potential enrollees.
- Letter mailed (n = 57,364).
Final classification categories:
- Potential enrollees (n = 15,982): test sample (n = 7,991) and validation sample (n = 7,991).
- Possibly potential enrollees (n = 13,763): test sample (n = 6,882) and validation sample (n = 6,881).
- Not potential enrollees (n = 31,785).
Overall Approach to the Analyses
We used individual-level data on the 15,982 potential enrollees in the MHTS and on the 13,763 possibly potential enrollees to estimate reduced-form models of the MHTS enrollment outcomes. Logistic regression was used to predict enrollment outcomes by regressing a 0-1 enrollment outcome dummy on a variety of personal characteristics of beneficiaries that are routinely available from Social Security administrative data files, as well as characteristics of the location of residence of the beneficiary that are publicly available from other data sources. A principal objective of this analysis was to provide information on enrollment in the MHTS that allowed comparisons of beneficiaries who enrolled with the larger group of beneficiaries who did not enroll. Such comparisons are important for considering the universe of beneficiaries to which one might appropriately generalize the outcome results from the MHTS.
Two different reduced-form models were estimated separately: one for potential enrollees and another for potential plus possibly potential enrollees. The two different models are based on two opposing assumptions about the source of differences between the two groups of beneficiaries. Combining the two groups assumes no effects of unobserved differences in the enrollment process between beneficiaries in each of the two groups. Estimation of a separate model for potential enrollees assumes that the unobserved factors that precluded possibly potential enrollees from speaking with MHTS personnel were not correlated with the unobserved factors that influenced potential enrollees' enrollment decisions. A third possible assumption is that these two sets of unobserved factors were correlated, resulting in a selection bias in estimates from the reduced-form models for potential enrollees. To explore that possibility, we also reestimated those models using a Heckman selectivity-correction approach and a probit enrollment regression. However, results of that analysis (available from the authors) failed to reject the null hypothesis of no selection bias.
Finally, although we focus on reduced-form models, we also present estimates (in the Appendix table) of a structural model that breaks the recruitment process down into the following three outcomes: a 0-1 dummy for being potential enrollees, a 0-1 dummy for potential enrollees attending the RIG meeting, and a 0-1 dummy for RIG meeting attendees enrolling as participants in the study. This analysis parallels the approach used in a recent study of enrollment in an SSA-sponsored randomized trial to promote work among SSI recipients—the New York WORKS Demonstration Project (Ruiz-Quintanilla and others 2005/2006).
Statistical Methods
To arrive at final regression estimates for our reduced-form enrollment models, we undertook a substantial effort in specification searching via exploratory regression analyses. This effort was necessitated by several factors. First, data on a large number of potentially relevant explanatory variables were available for our analyses, including a number of variables that were closely related in meaning to one another (for example, measures of recent preenrollment labor-market activity). Second, the dearth of existing literature directly relevant to our analyses meant that we had little in the way of prior empirical or conceptual guidance in distinguishing between relevant and irrelevant explanatory variables. Similarly, we had little prior evidence to guide us in terms of choosing the functional form for those continuous explanatory variables under observation.
An important consequence of using a substantial specification search with exploratory regressions is the problematic use of data from exploratory analyses to conduct valid hypothesis tests on the final models chosen in the specification search. Overfitting will result in p-values that are biased downward and an elevated risk of a type-I error.5 A widely used approach to resolve that difficulty and obtain valid statistical tests is to hold out a portion of data from the exploratory analyses and use that portion to validate the final models selected from the specification search (Studenmund 2011, 185–186; Kennedy 2008, chap. 5; Hilbe 2009, 286–290). We followed that approach in our analysis, using a two-step split-sample replication process in testing hypotheses and obtaining parameter estimates for the reduced-form models. We randomly divided our data into two halves, designating one half to serve as the “test” sample for our exploratory regressions and the other half as the “validation” sample.
Exploratory regressions estimated on the test-sample data included regressions restricted to potential enrollees as well as regressions including both potential and possibly potential enrollees. A large number of regressor variables were included in the initial exploratory regressions. Subsequent exploratory regressions included only selected regressors from the initial regressions, based on the signs, significance, and magnitudes of their coefficients in the initial regressions. This “testing-down” process continued until the final set of regressors was obtained. We discuss the results of those exploratory regressions and report the two “final” regression models (one for potential enrollees, and one for possibly potential plus potential enrollees) selected from this process. Then we report the results of reestimating these final regression models using only the data from the validation sample.
Several alternative logistic regression methods were used to obtain coefficient estimates: (1) regressions with separate site intercepts treated as regression parameters, (2) conditional logit regressions (with fixed site effects as incidental parameters), (3) regressions without site intercepts or fixed effects, (4) random effects regressions with random intercepts for each site, and (5) random effects regressions with random intercepts for each site and for each of 63 counties where study beneficiaries resided. All analyses were performed using the pseudo-maximum-likelihood algorithm in Stata (version 11), and all p-values for our coefficient and marginal effect estimates were robust (that is, they allowed for clustering by site). Coefficient estimates and two-tailed p-values for our regressors were very stable across all these methods. Results reported below are those obtained using method 1.6,7
Explanatory Variables
This section provides a discussion on variable selection, definitions, and data sources.
Conceptual and Practical Considerations in Explanatory Variable Selection
Because the MHTS intervention focused on IPS-SE services, our selection of potentially relevant regressors was based primarily on the view that a decision to enroll is positively related to a desire to increase work opportunities and earnings. That selection implied that factors identified in previous research as potential predictors (positive or negative) of labor supply and earnings would be related (positively or negatively) to the probability of enrollment in the MHTS. In addition, because the MHTS intervention also provided improved access to behavioral health treatment services, factors predictive of a demand (higher or lower) for this improved access should be related (positively or negatively) to enrollment probability. In some cases, both of these perspectives have similar implications for the expected positive or negative sign of an explanatory variable. For example, a DI beneficiary who is also receiving SSI payments presumably has less to gain from increased labor supply (because of a weaker work history and because of the reduction in SSI payments as his or her earnings increase). An individual receiving concurrent SSI/DI benefits also has greater access to behavioral health services because of Medicaid coverage; thus, one might expect receipt of SSI payments to negatively impact the probability of enrolling in the MHTS. By contrast, a variable such as “severity of mental disorder symptoms” might be expected to negatively impact expected earnings gains from the MHTS, but positively impact the demand for increased access to behavioral health treatments.8
Although these two perspectives suggest a large number of possible explanatory variables, available data on the target population of beneficiaries are restricted to the variables in both the Social Security administrative data sets and the linked data on the geographic area in which each beneficiary resides. Thus, our selection of beneficiary-level explanatory variables is confined primarily to demographic and diagnostic characteristics, information on benefit history, and only selected indicators of previous labor market–related activity that are captured in Social Security's administrative data.9
Variable Definitions and Data Sources
Data definitions and sources for selected explanatory variables used in our regression analyses are given in Table 1. (Brief descriptions of additional regressors in the exploratory regressions are given in the following section.) The principal data sources for our explanatory variables are two Social Security administrative databases: the Master Beneficiary Record file (MBR) and the Disability Control File (DCF). SSA maintains these files on all DI beneficiaries. The MBR was the principal source for variables describing individual beneficiary characteristics. Data from the DCF were used to define variables relating to beneficiaries' prerecruitment work activities.
Variable name | Definition | Source |
---|---|---|
Beneficiary characteristic | ||
Age/100 | Age in hundreds of days | MBR; RD |
Age/100^2 | Age/100 * Age/100 | MBR; RD |
Gender | = 1 for male; 0 for female | MBR |
Race | = 1 if black; 0 otherwise | MBR |
Mooddisdum | = 1 for beneficiary with an affective mood disorder; 0 otherwise | MBR; RD |
SPMD_primary | = 1 if primary diagnosis is for a severe and persistent mental disorder (SPMD); 0 otherwise | MBR; RD |
SPMD_second | = 1 if secondary diagnosis is for an SPMD; 0 otherwise | MBR; RD |
SPMD_either | = 1 if either a primary or secondary diagnosis is for an SPMD; 0 otherwise | MBR; RD |
Recruitment period | ||
Yr2006 | = 1 if recruited in calendar year (CY) 2006; 0 otherwise | SMS |
Yr2007 | = 1 if recruited in CY2007; 0 otherwise | SMS |
Yr2008 | = 1 if recruited in CY2008; 0 otherwise | SMS |
Exposure | # of days between contact date and site recruitment end date | SMS; RD |
Exposure^2 | Exposure × Exposure | SMS; RD |
Tract/block group sociodemographic | ||
Edu_bach | % in tract with more than a high school degree, by age and gender | CSF |
Edu_hs | % in tract with high school equivalent education, by age and gender, no bachelor's | CSF |
Edu_nohs | % in tract with less than a high school equivalent, by age and gender | CSF |
lnMedEarnings | Log of median 1999 earnings of all persons in block group aged 16 or older, by gender | CSF |
Residence type | ||
RepPayee | = 1 if address on file is for the representative payee; 0 otherwise | MBR |
POBox | = 1 if address is a PO Box; 0 otherwise | MBR |
lnDrivingdist | Log of driving distance to site (in miles) | MBR; SMS |
Recipiency history | ||
Monthsonrolls | Months on Disability Insurance (DI) rolls, based on recruitment date and MBR date of entitlement | MBR; RD |
Ssidum | = 1 if recipient of Supplemental Security Income (SSI); 0 otherwise | MBR |
Mrolls24nossi | = 1 if months on DI are < 24 and not a recipient of SSI; 0 otherwise | MBR; RD |
PIA | primary insurance amount | MBR; RD |
NHIStart | = 1 if not covered by Medicare at recruitment date; 0 otherwise | MBR; RD |
Prerecruitment date work-related activity | ||
ActiveT90dayspre | = 1 if had an active Ticket in the last 90 days before recruitment; 0 otherwise | DCF; RD |
ActiveTeverpre | = 1 if ever had an active Ticket before recruitment date; 0 otherwise | DCF; RD |
TWP10+pre | = 1 if beneficiary had trial work period (TWP) end date 10+ years before recruitment date; 0 otherwise | DCF; RD |
TWP5-10pre | = 1 if beneficiary had TWP end date 5–10 years before recruitment date; 0 otherwise | DCF; RD |
TWP0-5pre | = 1 if beneficiary had TWP end date 0–5 years before recruitment date; 0 otherwise | DCF; RD |
TWP0-3post | Beneficiary had a TWP end date 0–3 years after recruitment date; 0 otherwise a | DCF; RD |
Sqrt_Earn1_6pre | Square root of sum of beneficiary's self-reported earnings and self-employment net income 1–6 months before recruitment date; 0 if no report | DCF; RD |
Sqrt_Earn7_23pre | Square root of sum of beneficiary's self-reported earnings and self-employment net income 7–23 months before recruitment date; 0 if no report | DCF; RD |
NoEarnRpt1_6pre | No self-report of earnings or self-employment net income in 6 months before recruitment date = 1; 0 otherwise | DCF; RD |
Labor-market variable | ||
Unempcurrent | Unemployment rate in the county where the beneficiary lives, as of the month of the recruitment date | LAUS; RD |
Unempdelta | Unemployment rate in the county where the beneficiary lives 6 months before recruitment date | LAUS; RD |
SOURCE: Westat (2011). | ||
NOTES: CSF = Census Summary File (2000); DCF = Disability Control File; LAUS = Local Area Unemployment Statistics (Bureau of Labor Statistics); MBR = Master Beneficiary Record; RD = recruitment date; SMS = Survey Management System (Westat). | ||
a. A trial work period can extend over a period of 5 years; thus, an end date 3 years after the recruitment date implies a start date 2 years before the recruitment date. |
Many of the variables that used DCF or MBR data also incorporated information on the recruitment date that we assigned to each beneficiary. The assigned recruitment date was the date on which the recruitment letter was printed for the 55,097 beneficiaries (among the total 61,530 beneficiaries in the target population) for whom this date was available. For the remaining cases missing a recruitment letter date, we substituted the first available date from Westat's Study Management System in the following sequence: initial contact date (144 cases), follow-up contact date (27 cases), spoke to beneficiary date (1 case), and attempted contact date (2,095 cases). For the remaining 4,166 cases, we assumed that no recruitment letters were generated because they had no other dates in the system.10
MBR and DCF data did not contain information on two socioeconomic characteristics of beneficiaries—household income and education—missing for a substantial minority of beneficiaries. Therefore, we used the year-2000 version of the Census Summary Files (CSFs) to construct proxies for those variables.11,12 In addition, to test for the possible influences of local labor-market conditions, we used as explanatory variables county-level data—on unemployment and on employment (both as of the recruitment date and the 6 months that lagged)—from the Bureau of Labor Statistics (BLS) Local Area Unemployment Statistics (LAUS) program. The beneficiary's address from the MBR was used to match CSF and BLS/LAUS variables with corresponding counties or census tracts. The address from the MBR was also combined with demonstration site address information from Westat's Survey Management System to compute a variable for driving distance to the demonstration site; this was done to test the hypothesis that distance (as a proxy for travel time and costs) would be negatively related to the probability of enrolling in the MHTS.
Values for the means and standard deviations for the explanatory variables are shown in Table 2 for potential enrollees and possibly potential enrollees.13 (Note that 234 potential enrollees and 361 possibly potential enrollees were excluded from the table14 because of (1) missing values for at least one of the variables in the table, (2) MBR data indicating a date of death prior to either October 2006 or the beneficiary's recruitment date, or (3) coded values that were clearly in error; examples of this were negative values for the time-to-recruit variable and values outside the 0-1 interval for the census-tract fraction of persons with at least some college education.)
Variable name | Potential enrollees only (n = 15,748) | Possibly potential enrollees only (n = 13,402) | ||
---|---|---|---|---|
Mean | Standard deviation | Mean | Standard deviation | |
Beneficiary characteristic | ||||
Age/100 x (100/365) (= age in years) | 45.9 | 7.4 | 44.3 | 8.2 |
Gender (= 1 if male) | 0.449 | 0.497 | 0.490 | 0.500 |
Race (= 1 if black) | 0.243 | 0.429 | 0.273 | 0.446 |
Mooddisdum | 0.684 | 0.465 | 0.678 | 0.467 |
SPMD_primary | 0.727 | 0.446 | 0.660 | 0.474 |
SPMD_second | 0.276 | 0.447 | 0.251 | 0.433 |
SPMD_either | 0.729 | 0.444 | 0.661 | 0.473 |
Recruitment period | ||||
Yr2006 | 0.088 | 0.283 | 0.042 | 0.200 |
Yr2007 | 0.504 | 0.500 | 0.301 | 0.459 |
Yr2008 | 0.408 | 0.491 | 0.657 | 0.475 |
Exposure | 279.2 | 168.7 | 202.8 | 148.8 |
Tract/block group sociodemographic | ||||
Edu_bach | 0.264 | 0.192 | 0.262 | 0.201 |
Edu_hs | 0.548 | 0.151 | 0.532 | 0.158 |
Edu_nohs | 0.188 | 0.153 | 0.206 | 0.168 |
Median earnings (anti log of lnMedEarnings) | 25,706 | 9,179 | 25,051 | 9,366 |
Residence type | ||||
RepPayee | 0.161 | 0.367 | 0.267 | 0.442 |
POBox | 0.037 | 0.190 | 0.062 | 0.241 |
Driving distance (antilog of lnDrivingdist) | 11.42 | 12.42 | 12.12 | 20.61 |
Recipiency history | ||||
Monthsonrolls | 118.7 | 78.0 | 110.9 | 77.1 |
Ssidum | 0.191 | 0.393 | 0.223 | 0.416 |
Mrolls24nossi | 0.036 | 0.186 | 0.052 | 0.223 |
PIA | 8,501 | 3,563 | 8,169 | 3,468 |
NHIStart | 0.050 | 0.218 | 0.063 | 0.243 |
Prerecruitment date work-related activity | ||||
ActiveT90dayspre | 0.046 | 0.210 | 0.044 | 0.205 |
ActiveTeverpre | 0.070 | 0.255 | 0.059 | 0.235 |
TWP10+pre | 0.063 | 0.242 | 0.058 | 0.233 |
TWP5-10pre | 0.054 | 0.225 | 0.052 | 0.222 |
TWP0-5pre | 0.049 | 0.217 | 0.071 | 0.258 |
TWP0-3post | 0.009 | 0.096 | 0.009 | 0.096 |
Sqrt_Earn1_6pre | 236 | 1,465 | 455 | 2,241 |
Sqrt_Earn7_23pre | 885 | 4,047 | 1,464 | 6,281 |
NoEarnRpt1_6pre | 0.929 | 0.256 | 0.897 | 0.304 |
Labor-market variable | ||||
Unempcurrent | 4.59 | 1.13 | 4.83 | 1.32 |
Unempdelta | 4.40 | 1.06 | 4.43 | 0.99 |
SOURCE: Westat (2011). | ||||
NOTES: Only data for persons included in the logit regressions are included in this table. PIA = primary insurance amount; SPMD = severe and persistent mental disorder; SSI = Supplemental Security Income; TWP = trail work period. |
Possibly potential enrollees show smaller values (than potential enrollees) for the time-to-recruit variable, and relatively more of them had recruitment dates in 2008; thus, it is likely that recruitment activities were terminated more rapidly for that group than they were for the potential enrollees. Possibly potential enrollees also reported slightly higher mean earnings to SSA in the prerecruitment period (though some of that differential was due to the influence of a few very large earnings figures), a much higher rate of representative payees (26.7 percent versus 16.1 percent), and a larger right tail for the distribution of distance values.
Reduced-Form Enrollment Regression Results
In this section, we discuss in detail our exploratory regressions in addition to our validation-sample regressions.
Exploratory Regressions
We began our empirical investigations by estimating a large number of exploratory logistic regressions on two samples: (1) a test sample composed of a 50.0 percent random sample of potential enrollees and possibly potential enrollees and (2) a subset of this test sample that only included the potential enrollees. The exploratory regressions included all of the explanatory variables (shown in Table 1) that were used in at least some of our analyses, with almost all of those variables included in the initial exploratory regressions.15
Coefficients and standard errors for the exploratory regressions using our test-sample data were estimated using a variety of methods including maximum likelihood with separate site intercepts, conditional (fixed effects) maximum likelihood, maximum likelihood with clustering of errors (by site), and random-effects logistic regressions (with random intercepts for each site). Variables were retained in the final exploratory regressions based on their p-values and (in select cases) the consistency of the regression results with prior expectations, evidence, or theory.16
Because some qualitative results (that is, coefficient signs and significance) for individual explanatory variables varied between the exploratory regressions for potential enrollees and those for potential plus possibly potential enrollees, our general approach was to retain variables that met our criteria. Variables with estimated coefficients having a two-tailed p-value of approximately 0.10 or less in at least one of the two test-sample regressions were included in the validation-sample regressions.
Table 3 provides results from the final versions of the exploratory regressions on the test sample: Potential enrollees are shown in model 1 and potential plus possibly potential enrollees are shown in model 2. All other variables noted earlier in this section that did not meet our inclusion criteria were dropped; several variables were dropped because of stronger results for other variables that were close substitutes (for example, per capita income was dropped in favor of median earnings, and Edu_hs and Edu_nohs were dropped in favor of Edu_bach). The results reported in Table 3 for each variable show the average marginal effects (that is, the change in predicted probability of enrollment in response to a marginal change in the value of each variable).17 All regressions in the table were estimated with site-specific dummy variables, and standard errors allowed for clustering by site.
Variable name | Test sample | Validation sample | ||||||
---|---|---|---|---|---|---|---|---|
Potential enrollees only (n = 7,815): model 1 | Potential + possibly potential enrollees (n = 14,513): model 2 | Potential enrollees only (n = 7,933): model 3 | Potential + possibly potential enrollees (n = 14,637): model 4 | |||||
dy/dx | P > z | dy/dx | P > z | dy/dx | P > z | dy/dx | P > z | |
Age/100 a | -0.0008 | <0.001 | -0.0003 | 0.006 | -0.0003 | 0.112 | 7.7E-07 | 0.994 |
Gender (male = 1) | 0.0256 | 0.002 | 0.0084 | 0.066 | 0.0150 | 0.052 | 0.0059 | 0.199 |
Race (black = 1) | 0.0331 | <0.001 | 0.0151 | 0.008 | 0.0301 | <0.001 | 0.0188 | <0.001 |
Yr2006 | 0.0277 | 0.076 | 0.0088 | 0.312 | 0.0264 | 0.040 | 0.0080 | 0.229 |
Exposure a | 0.0001 | 0.001 | 0.0001 | <0.001 | 0.0001 | 0.045 | 0.0001 | <0.001 |
Monthsonrolls | -0.0002 | 0.001 | -0.0001 | 0.002 | -0.0002 | <0.001 | -0.0001 | <0.001 |
Ssidum | -0.0226 | 0.083 | -0.0123 | 0.073 | -0.0212 | 0.033 | -0.0138 | 0.023 |
RepPayee | -0.0559 | <0.001 | -0.0514 | <0.001 | -0.0634 | <0.001 | -0.0519 | <0.001 |
lnDrivingdist | -0.0130 | 0.015 | -0.0095 | 0.001 | -0.0123 | 0.019 | -0.0077 | 0.044 |
Edu_bach | 0.0628 | 0.091 | 0.0304 | 0.141 | -0.0142 | 0.491 | -0.0068 | 0.560 |
lnMedEarnings | -0.0431 | 0.017 | -0.0192 | 0.031 | -0.0069 | 0.553 | 0.0015 | 0.832 |
ActiveT90dayspre | 0.0332 | 0.059 | 0.0147 | 0.114 | 0.0162 | 0.468 | 0.0079 | 0.515 |
ActiveTeverpre | 0.0975 | <0.001 | 0.0581 | <0.001 | 0.0796 | <0.001 | 0.0539 | <0.001 |
TWP10+pre | 0.0460 | 0.030 | 0.0249 | 0.028 | 0.0577 | <0.001 | 0.0333 | <0.001 |
TWP5-10pre | 0.0391 | 0.002 | 0.0201 | 0.004 | 0.0145 | 0.349 | 0.0081 | 0.381 |
TWP0-5pre | 0.0360 | 0.114 | 0.0227 | 0.069 | 0.0362 | 0.020 | 0.0148 | 0.116 |
TWP0-3post | 0.1363 | <0.001 | 0.0732 | <0.001 | 0.0807 | <0.001 | 0.0541 | <0.001 |
Sqrt_Earn1_6pre | -0.0017 | <0.001 | -0.0013 | <0.001 | -0.0022 | <0.001 | -0.0014 | <0.001 |
Sqrt_Earn7_23pre | 0.0004 | 0.107 | 0.0002 | 0.137 | 0.0001 | 0.459 | 9.6E-06 | 0.920 |
NoEarnRpt1_6pre | -0.0771 | 0.002 | -0.0445 | 0.002 | -0.1018 | <0.001 | -0.0601 | <0.001 |
SOURCE: Westat (2011). | ||||||||
NOTES: All regressions include site-specific dummy variables. All p-values allow for clustering of errors by site.
SSI = Supplemental Security Income; TWP = trial work period.
|
||||||||
a. Marginal effects are based on a combination of regression coefficients for linear and squared terms. |
The results of the exploratory regressions generally conform to prior expectations based on the enhancing-earnings view of the MHTS, articulated earlier in the Explanatory Variables section. The negative marginal effect of age (-0.0008) is consistent with the thesis that the enrollees' expected payoff period from efforts to increase their work opportunities declines with age (because of the approach of retirement and the increasing risk of further declines in health). Demographic variables relating to gender and race show directions of marginal effects (positive for males and for blacks) that are consistent with prior findings from the labor economics literature about the determinants of labor supply (Altonji and Blank 1999; Pencavel 1986; Killingsworth and Heckman 1986). The negative effects of area median earnings and education levels are more difficult to interpret because those variables could be predictive of both positive market-opportunity wage effects and negative income effects on enrollees' efforts to increase their work opportunities.
A number of other indicator variables from Social Security's administrative data also showed effects on enrollment probabilities consistent with this enhancing-earnings perspective. The negative coefficients for enrollees' SSI receipt and for having a representative payee may reflect poorer future earnings prospects because of more limited work experience and lower expected market wages for those enrollees. Dummy variables relating to prior work (trial work period, or TWP) activities and prior use of Ticket to Work (TTW) opportunities all have positive marginal effects. The pattern of results indicates that persons with the most recent involvement in trial work or return-to-work efforts have the highest probability of enrollment. For example, based on the figures in model 1, a person with an active TTW within 90 days of the recruitment date has a predicted enrollment probability that is roughly 13.1 percent higher (= 3.3 + 9.8) than that for a person with no prior active TTW. Similarly, a person with a TWP whose end date was less than 3 years after the recruitment date has a predicted enrollment probability that is roughly 13.6 percent higher than that for a person without such a TWP. Results for prerecruitment self-reported earnings show an interesting pattern, in that the presence of earnings in the 6 months or less prior to the recruitment date tends to reduce the probability of enrollment, while the presence of earnings in more than 6 months prior to recruitment tends to increase the probability of enrollment; this suggests that persons with very recent self-reported earnings may feel less need to increase their opportunities for earnings. On the other hand, persons who did not file an earnings report with SSA covering any of the 6 months prior to recruitment were also less likely to enroll. Most of those individuals presumably had virtually no earnings in the recent past; therefore, they had not received any requests from SSA to verify their earnings history. (Others may have had nonreported “under-the-table” earnings and preferred not moving into “regular” jobs with reported income.)
The estimated negative marginal effect for months on the rolls indicates that on average, each additional month reduces the probability of enrollment by 0.02 percent. This finding is consistent with the presumption that as beneficiaries remain on the DI rolls for longer periods, they are less likely to pursue opportunities to increase their earnings and perhaps leave the rolls (Mashaw and Reno 1996).
The negative marginal effect of distance to the site is expected, based on the assumption that the time and money cost to the enrollees of participating in treatment is positively related to distance. The positive effect of the time-to-recruit variable is as expected; persons with a longer time window for completing the recruitment process are more likely to complete the process. This may also explain the positive year-2006 effect, although it may also be related to the correlation between earlier recruitment efforts and beneficiaries' residence in the initial catchment areas for the sites.
Validation-Sample Regressions
Corresponding estimates for marginal effects from the validation-sample regressions are reported in Table 3: in model 3 for potential enrollees and in model 4 for potential plus possibly potential enrollees. (Coefficient estimates are available from the authors upon request.) The p-values for a number of variables substantially exceeded those for the test samples, as would be expected when looking at the large number of regressions run on the test samples, which imparts an upward bias to the z-scores of the marginal-effect estimates. On the whole, however, these results generally parallel—in both sign and magnitude—the test-sample results, and the p-values for the marginal effects are generally quite significant. The main exceptions are the two year-2000 Census Summary File variables, the dummy for an active Ticket within 90 days of the recruitment date, the dummy for a TWP ending 5 to 10 years prior to recruitment, and the effect of prior earnings self-reported to SSA more than 6 months before the recruitment date.
Following Hilbe (2009), we assessed the goodness of fit of our logistic regressions using the Hosmer-Lemeshow test procedure. In particular, we placed our observations in ascending order of predicted enrollment probability, divided the observations into deciles, and compared the mean predicted enrollment probability for each decile with the actual fraction enrolled within that decile. The test statistic is an χ² with (10,2) degrees of freedom; higher p-values indicate a better model fit (and failure to reject the null hypothesis that the columns of probabilities were drawn from the same distribution).
Test results from our goodness-of-fit analysis are reported in Table 4 for each of the four regression models shown in Table 3. For both the exploratory regressions with the test samples and the regressions with the validation samples, the mean predicted enrollment probabilities within each decile is fairly close to the actual fraction enrolled. Thus, the p-values based on the deciles (p(10)) are clearly not significant, suggesting that the regression models are a good fit to the actual data. Because the results of the Hosmer-Lemeshow test may vary with the number of groups used, we also reported the results of the test using quintiles (p(5)) and using 20 ordered groups of observations (p(20)). In all cases, the p-values are consistently far above any level that would indicate rejection of the null hypothesis of a significant fit of the model to the data.
Deciles a of ordered groups of observations | Final test-sample exploratory regressions | Validation-sample regressions | ||||||
---|---|---|---|---|---|---|---|---|
Potential enrollees only | Potential + possibly potential enrollees | Potential enrollees only | Potential + possibly potential enrollees | |||||
Mean prediction of enrollment probability | Actual enrollment fraction | Mean prediction of enrollment probability | Actual enrollment fraction | Mean prediction of enrollment probability | Actual enrollment fraction | Mean prediction of enrollment probability | Actual enrollment fraction | |
1 | 0.051 | 0.049 | 0.020 | 0.021 | 0.049 | 0.052 | 0.018 | 0.014 |
2 | 0.071 | 0.070 | 0.030 | 0.028 | 0.069 | 0.053 | 0.029 | 0.028 |
3 | 0.086 | 0.090 | 0.038 | 0.039 | 0.082 | 0.093 | 0.037 | 0.038 |
4 | 0.100 | 0.085 | 0.046 | 0.043 | 0.095 | 0.088 | 0.044 | 0.038 |
5 | 0.114 | 0.119 | 0.055 | 0.052 | 0.109 | 0.102 | 0.051 | 0.050 |
6 | 0.129 | 0.119 | 0.065 | 0.066 | 0.125 | 0.141 | 0.060 | 0.064 |
7 | 0.147 | 0.148 | 0.077 | 0.078 | 0.142 | 0.141 | 0.072 | 0.072 |
8 | 0.173 | 0.174 | 0.094 | 0.091 | 0.165 | 0.160 | 0.089 | 0.099 |
9 | 0.216 | 0.251 | 0.122 | 0.130 | 0.201 | 0.209 | 0.118 | 0.128 |
10 | 0.364 | 0.347 | 0.233 | 0.234 | 0.318 | 0.315 | 0.216 | 0.204 |
p(5) b | 0.703 | . . . | 0.866 | . . . | 0.570 | . . . | 0.568 | . . . |
p(10) b | 0.299 | . . . | 0.982 | . . . | 0.438 | . . . | 0.489 | . . . |
p(20) b | 0.448 | . . . | 0.977 | . . . | 0.558 | . . . | 0.772 | . . . |
SOURCE: Westat (2011). | ||||||||
NOTE: . . . = not applicable. | ||||||||
a. Deciles are based on the predicted probability for each model. | ||||||||
b. p(n) is the p-value for the Hosmer-Lemeshow χ² test with n groups. |
Discussion
In this section, we compare the recruitment results of the Mental Health Treatment Study with those of other work-incentive programs. We also discuss the implications of our findings and then present concluding comments.
Comparisons with Results from Previous Programs
As noted in the Recruitment and Enrollment Processes section, the MHTS recruited 2,238 enrollees; that represents 14.0 percent of the 15,982 potential enrollees and 7.5 percent of the 29,745 possibly potential and potential enrollees (combined), but only 5.9 percent of 37,693 beneficiaries who were not excluded because of ineligibility or Westat's Survey Management System codes of “no letter mailed” or “could not locate” (see the flowchart discussed and presented in the Recruitment Outcomes section). These percentages are substantially better than enrollment rates from previous SSA recruitment efforts toward randomized trial interventions, such as Project NetWork (4.5 percent) and the New York WORKS project (2.4 percent for members of the two treatment groups with valid addresses).18 The MHTS recruitment rate is also well above the enrollment rate observed for the TTW program (1.8 percent as of December 2005) for the 13 phase-1 states participating in the initial implementation (for further details, see Stapleton and others (2008, Executive Summary)).19 Because those programs defined their target groups differently from the MHTS, had varying time constraints on the recruitment process, and varying approaches to recruiting participants, such differences in enrollment rates are not entirely unexpected.20
Qualitative findings about several significant predictors of enrollment in those earlier interventions, however, show similarities to the results of this study. Evaluations of Project NetWork (Burstein, Roberts, and Wood 1999) and New York WORKS (Ruiz-Quintanilla and others 2005/2006) both found strong positive effects of recent work experience and earnings. The univariate analysis in the TTW evaluation reported that DI beneficiaries who entered an extended period of eligibility (EPE) were much more likely to participate by 2005, and that the enrollment rate was highest for those who most recently entered an EPE (Stapleton and others 2008, chap. 3). This parallels our finding that beneficiaries who had any TWP were more likely to enroll in the MHTS, and that enrollment rates were highest among those who entered a TWP most recently.21
These previous studies also examined enrollment rates by months on the disability rolls, with varying results. The TTW evaluation found a positive relationship over months 0 to 60 and then some evidence of a gradual enrollment decline as the number of months increased (Stapleton and others 2008, chap. 3). The Project NetWork study found the highest enrollment rates among persons receiving disability benefits from 2 to 5 years (Burstein, Roberts, and Wood 1999). New York WORKS found higher enrollment rates for persons on SSI for either less than 24 months or more than 96 months. This contrasts with the monotonically negative relationship we reported earlier. Similarly, Project NetWork reported evidence that individuals receiving concurrent SSI/DI benefits had the highest enrollment rates, and SSI recipients had the lowest. Similarly, and in contrast to our results reported earlier, the TTW evaluation also found in univariate analyses that recipients of concurrent benefits had higher enrollment rates than DI-only beneficiaries. However, that result was not confirmed in multivariate analyses on a more limited sample of beneficiaries.22
Qualitative results of the three previous studies also did not always correspond closely with our findings on demographic and socioeconomic characteristics. Age showed more consistently negative effects on enrollment rates in the Ticket to Work and New York WORKS studies, but the rate was highest for the group aged 31–40 in the Project NetWork study. None of the studies reported strong evidence of gender or race differences in enrollment. Only the Ticket to Work and Project NetWork studies reported evidence of effects of educational attainment on enrollment; both studies found those effects to be positive. Only Project NetWork reported a comparison of rates by household income, which did not show differences.
Given the differences in the target populations and recruitment methods of other studies relative to the MHTS, some differences in the results of other work-incentive efforts compared with our own findings are to be expected. In addition, differences based on multiple regression results in the New York WORKS and Ticket to Work studies may also reflect differences in the sets of included explanatory variables compared with the present study.
Study Implications
A number of our explanatory variables drawn from Social Security administrative data files are strong predictors of enrollment. Thus, in considering the results of the MHTS intervention, it is important to recognize that the MHTS participants differed from the overall target population of beneficiaries recruited for the study. In particular, relative to the overall target population, MHTS participants were more likely to be beneficiaries with histories of recent earnings (as indicated by preenrollment earnings reports and/or recent TWPs) or an active Ticket. Conversely, MHTS participants were less likely to be beneficiaries who were nonwhite, on SSI, and/or on the DI rolls for a longer period of time.
Our results may be of interest to SSA for possible targeting of subsequent efforts by the agency either to replicate the MHTS experiment or to proceed with actually implementing the MHTS intervention (that is, providing the package of MHTS treatment services to additional beneficiaries with schizophrenia or affective disorders). Specific targeting strategies would, of course, depend on the objectives of that targeting. For example, to reach a given enrollment target number, SSA could target its subject recruitment efforts to specific beneficiary groups with higher probabilities of enrollment in order to reduce recruitment costs. There may be a cost trade-off, however, between using targeting variables that produce very high enrollment rates but small numbers of enrollees (because they exclude a large fraction of all beneficiaries), versus targeting variables that yield lower enrollment rates but exclude fewer beneficiaries.
Information that could be used to assess alternative targeting strategies—aimed at increasing the enrollment rates for our validation samples—is presented in Table 5. Results are presented in descending order of predicted enrollment rates. As indicated in the first row of the table, among all the indicators that we tested, targeting recruitment to potential enrollees who had an active Ticket at any time prior to enrollment yields the highest mean predicted enrollment probability of 25.3 percent, but yields only 152 predicted enrollees because the number of potential enrollees with an active Ticket is relatively small. Strategies based on the presence of either one of two characteristics can increase the number of predicted enrollees with only modest drops in the enrollment rate. Thus, the second row of the table shows that using either the Ticket criterion or the criterion for the most recent trial work period yields almost the same predicted enrollment probability of 22.2 percent, while increasing the predicted enrollment number to 216. As noted in the sixth row of the table, using the same Ticket criterion or any trial work period yields a predicted enrollment probability of 18.8 percent, while increasing the predicted enrollment number to 331. Similar results emerge when we consider potential plus possibly potential enrollees (see the last three columns of Table 5).
Selection criterion | Potential enrollees only | Potential + possibly potential enrollees | ||||
---|---|---|---|---|---|---|
Predicted enrollees (%) | Number in sample | Predicted enrollees (n) | Predicted enrollees (%) | Number in sample | Predicted enrollees (n) | |
Ever had an active Ticket to Work | 25.3 | 601 | 152 | 15.4 | 986 | 152 |
Ever had active Ticket or trial work period end date 5 years before to 3 years after enrollment | 22.2 | 975 | 216 | 11.8 | 1,830 | 216 |
Had trial work period end date 5 years before to 3 years after enrollment | 20.0 | 449 | 90 | 9.1 | 990 | 90 |
Any earnings report filed (1–6 months before enrollment) | 19.2 | 583 | 112 | 8.9 | 1,264 | 112 |
Reported possible earnings (1–6 months before enrollment) | 19.0 | 579 | 110 | 8.8 | 1,255 | 110 |
Ever had active Ticket or any trial work period | 18.8 | 1,758 | 331 | 10.1 | 3,277 | 333 |
Reported possible earnings (1–23 months before enrollment) | 18.3 | 1,078 | 197 | 8.6 | 2,279 | 197 |
Reported possible earnings (7–23 months before enrollment) | 18.1 | 1,004 | 181 | 8.5 | 2,135 | 181 |
Ever had a trial work period | 17.6 | 1,377 | 242 | 9.1 | 2,655 | 242 |
Had trial work period ending date 5 years or more before enrollment | 16.4 | 928 | 152 | 9.1 | 1,665 | 152 |
Ever had active Ticket or months on rolls < median | 15.7 | 4,302 | 674 | 8.2 | 7,838 | 645 |
Ever had active Ticket or InDrivingdist < median | 15.3 | 4,292 | 655 | 8.6 | 7,852 | 676 |
Reported possible earnings (1–23 months before enrollment) or months on rolls < median | 15.2 | 4,390 | 668 | 7.8 | 8,178 | 639 |
Months on rolls < median or had any trial work period | 15.2 | 4,855 | 738 | 7.9 | 8,950 | 709 |
Any earnings report filed (1–6 months before enrollment) or months on rolls < median | 15.2 | 4,202 | 638 | 7.8 | 7,795 | 608 |
Months on rolls < median or trial work period ending date 5 years before to 3 years after enrollment | 15.1 | 4,088 | 618 | 7.8 | 7,554 | 587 |
Months on rolls < median a | 15.0 | 3,965 | 595 | 7.7 | 7,281 | 561 |
Reported possible earnings (1–23 months before enrollment) or InDrivingdist < median | 14.9 | 4,541 | 678 | 8.1 | 8,542 | 696 |
InDrivingdist < median or trial work period ending date 5 years before to 3 years after enrollment | 14.8 | 4,212 | 625 | 8.2 | 7,859 | 647 |
Any earnings report file (1–6 months before enrollment) or InDrivingdist < median | 14.8 | 4,264 | 631 | 8.2 | 7,988 | 654 |
InDrivingdist < median b | 14.6 | 3,966 | 579 | 8.3 | 7,318 | 605 |
Does not have a RepPayee | 14.4 | 6,661 | 961 | 8.3 | 11,604 | 961 |
Is not a dual beneficiary | 13.9 | 6,452 | 898 | 7.7 | 11,697 | 898 |
Total validation-sample mean | 13.6 | 7,933 | 1,075 | 7.3 | 14,637 | 11 |
SOURCE: Westat (2011). | ||||||
a. The median months on the rolls for potential enrollees is 98; for potential + possibly potential enrollees, it is 92. | ||||||
b. The median log for potential enrollees is 2.278907; for potential + possibly potential enrollees, it is 2.353563. |
Other targeting strategies could be devised based on other objectives. For example, our results suggest that additional recruitment efforts would be needed to compensate for lower enrollment rates among nonwhites, women, and/or SSI recipients. Implementation in target geographic areas defined by proximity to intervention sites, as done in the MHTS, might suggest using our results to identify potential target sites within geographic areas with large numbers of potential enrollees. Finally, if information were also available on the characteristics of beneficiaries most likely to be positively impacted by the intervention (for example, in terms of returning to work), it could be combined with results presented here to design a recruitment strategy that accounts for these differential intervention impacts as well as differential recruitment costs.
Concluding Comments
In summary, as a result of the MHTS recruitment efforts, we observed participation rates of 5.9, 7.5, and 14.0 percent, depending on whether or how much a beneficiary had an opportunity to know about the study. The most conservative interpretation suggests an enrollment rate between 5.9 and 7.5 percent. However, it is reasonable to assume that some portion of the possibly potential enrollees were never informed about the study, and, therefore, do not belong in the denominator, which then suggests a less conservative estimate between 7.5 and 14.0 percent. Regardless of which estimate is the most accurate, they are all above the rate that might be expected based on previous experience. Moreover, we found that further targeting of beneficiary groups (based on routinely available administrative data) could increase this rate even further. (Our results suggest an upper bound approaching 25.0 percent.)
It could be argued that enrollment rates for a randomized trial such as the MHTS may in fact be lower than enrollment rates for a comparable service provided without randomization. If beneficiaries are influenced to enroll or not enroll, based at least in part on subjective calculations of expected costs and benefits of participation, one might expect randomization per se to have a negative impact on the enrollment rate because the enrolling beneficiaries would not be able to count on receiving the potential benefits that the intervention offered. This seems a relevant concern in the case of the MHTS because the preenrollment requirement of attending an RIG meeting meant that the costs of participation were nonnegligible.
Finally, the success of our enrollment efforts could have been enhanced even further by using data on a number of other individual characteristics of beneficiaries that were not available in the current study. These data include more detailed information on prior employment status and earnings as well as additional information on medical comorbidities beyond what is available in the MBR.
Appendix: Structural Equation Estimates
Structural equation models of a multistage recruitment process can provide potentially useful information—at a more detailed level than reduced-form models—about the influence of a variety of factors on the enrollment process. This analytical approach is particularly interesting when it provides evidence that the patterns of influence vary considerably across the recruitment stages. Ruiz-Quintanilla and others (2005/2006) presented an interesting analysis of the multistage recruitment process for the New York WORKS project using a set of structural logit regressions. In that case, four steps in the process were modeled: (1) having a valid address for receiving the recruitment letter, (2) returning a response card, (3) indicating an interest in participating in the project, and (4) enrolling in the project. Each step was modeled as a separate logit regression, and independence of unobservables across the four regressions was assumed.
We applied the same general approach in our analysis, modeling a sequence of three outcomes: (1) having a contact with the MHTS recruiter (and thus being classified as a potential enrollee), (2) attending at least one RIG meeting, and (3) enrolling in the MHTS. The corresponding three logit regressions were estimated on all study beneficiaries (that is, with test and validation samples combined). Explanatory variables were the same as those shown in Table 1.
Results of these regressions are reported in the Appendix table. In examining those results, bear in mind that the rate at which each recruitment stage included beneficiaries for the following stage varied considerably. Thus, of the 29,150 potential and possibly potential enrollees in this analysis, 54.0 percent (15,748) were contacted by recruiters, but of that group only 23.8 percent (3,753) actually attended an RIG meeting. Finally, of those 3,753 cases, more than half (2,206, or 58.8 percent) enrolled in the MHTS. Thus, the problem of attracting potential enrollees to an RIG meeting appears to have been a particular challenge for the recruiters.
Variable name | Possibly potential enrollees (n = 29,150): model 1— potential enrollee dummy | Potential enrollees (n = 15,748): model 2— RIG meeting dummy | Attended RIG meeting (n = 3,753): model 3— enrolled dummy | |||
---|---|---|---|---|---|---|
Coefficient | P > z | Coefficient | P > z | Coefficient | P > z | |
Age/100 | 0.0108 | 0.056 | 0.0188 | 0.033 | -0.0077 | 0.555 |
Age/100^2 | -1.7E-05 | 0.360 | -0.0001 | 0.010 | 1.9E-05 | 0.659 |
Gender (male = 1) | -0.1421 | <0.001 | 0.1853 | <0.001 | 0.0549 | 0.544 |
Race (black = 1) | -0.0344 | 0.386 | 0.4309 | <0.001 | -0.1875 | 0.042 |
Yr2006 | -0.2850 | 0.080 | 0.2322 | 0.083 | 0.0927 | 0.571 |
Yr2008 | -0.1847 | 0.438 | . . . | . . . | . . . | . . . |
Exposure | 0.0018 | 0.418 | 0.0033 | 0.001 | -0.0002 | 0.887 |
Exposure^2 | 3.5E-07 | 0.899 | -4.0E-06 | 0.011 | 3.1E-07 | 0.850 |
Monthsonrolls | 0.0003 | 0.268 | -0.0021 | <0.001 | -0.0008 | 0.108 |
Ssidum | -0.0614 | 0.156 | -0.0904 | 0.085 | -0.1888 | 0.027 |
RepPayee | -0.6353 | <0.001 | -0.4044 | <0.001 | -0.3381 | 0.002 |
Mooddisdum | -0.0764 | 0.006 | . . . | . . . | . . . | . . . |
lnDrivingdist | -0.0890 | 0.115 | -0.1154 | 0.001 | -0.0267 | 0.502 |
Edu_bach | -0.1438 | 0.373 | 0.1218 | 0.425 | 0.2038 | 0.478 |
Unempcurrent | 0.0381 | 0.686 | . . . | . . . | . . . | . . . |
Unempdelta | -0.1884 | 0.099 | . . . | . . . | . . . | . . . |
lnMedEarnings | 0.1735 | 0.199 | -0.1759 | 0.017 | -0.1385 | 0.392 |
ActiveT90dayspre | -0.0507 | 0.610 | 0.0879 | 0.456 | 0.3290 | 0.003 |
ActiveTeverpre | 0.3299 | <0.001 | 0.7858 | <0.001 | 0.1892 | 0.150 |
TWP10+pre | 0.0107 | 0.850 | 0.3754 | <0.001 | 0.2525 | 0.228 |
TWP5-10pre | -0.0435 | 0.550 | 0.2761 | <0.001 | -0.0188 | 0.921 |
TWP0-5pre | -0.0492 | 0.605 | 0.2565 | 0.015 | 0.2791 | 0.175 |
TWP0-3post | 0.1282 | 0.286 | 0.7775 | <0.001 | 0.7366 | 0.003 |
Sqrt_Earn1_6pre | -0.0076 | <0.001 | -0.0151 | <0.001 | -0.0090 | 0.028 |
Sqrt_Earn7_23pre | -0.0014 | 0.015 | 0.0032 | 0.003 | -0.0004 | 0.868 |
NoEarnRpt1_6pre | -0.0706 | 0.458 | -0.6580 | <0.001 | -0.5306 | 0.004 |
SOURCE: Westat (2011). | ||||||
NOTES: All regressions include site-specific dummy variables. All p-values allow for clustering of errors by site.
SSI = Supplemental Security Income; TWP = trial work period; . . . = not applicable.
|
Thus, it is interesting that the logit results in the table show a somewhat different pattern in model 2 compared with model 1 and a very different pattern in model 2 compared with model 3. When looking at the differences between model 1 and model 2, we see a much larger number of highly significant coefficients in the latter model. On the other hand, comparing model 2 with model 3, we see far fewer significant coefficients in model 3. This pattern of results has interesting implications for future MHTS-like efforts. For example, the much stronger negative coefficient for the distance variable in model 2 suggests that outreach efforts such as holding RIG meetings at multiple locations within each site's catchment area may increase recruiting success.
In considering this or other strategies for increasing recruitment success, it is also important to note that the potential enrollees who did not attend an RIG meeting were almost as numerous as the beneficiaries who did not make contact with the recruiter (that is, possibly potential enrollees who did not become potential enrollees). This fact also argues for focusing on the results of model 2 in assessing implications of our analysis for improving recruitment outcomes.23
Notes
1 The figures cited here are from SSA (2012, Table 24).
2 See, for example, Bond, Drake, and Becker (2008); Campbell, Bond, and Drake (2009); and Burns and others (2009).
3 See Drake and others (2013); Hall and others (2003); Wang, Berglund, and Kessler (2000); Lehman, Steinwachs, and the co-investigators of the PORT Project (1998).
4 See Frey and others (2008) and Westat (2011, chaps. 1–3) for further details on the design and implementation of the MHTS. Intervention results are presented in Westat (2011) and in Drake and others (2013).
5 As Studenmund (2011, 185) explains, “our typical statistical tests have little meaning if the new hypothesis is tested on the data set that was used to generate it.”
6 When unobserved site-specific errors are correlated with regressors, coefficient estimates for the regressors are consistent in method 1 only as Ti (= the number of observations in the “ith” site) goes to infinity (Cameron and Trivedi 2010, 627). However, several studies (Greene 2004; Wright and Douglas 1975) indicate that small-sample bias in our estimates should be negligible because the Ti s in our data are in the range of 61 to 853, with a median of 320 cases for our smallest study sample (potential beneficiaries in the validation sample). Estimates from method 2 are consistent regardless of the value of Ti (Cameron and Trivedi 2010, 627).
7 We preferred methods 1 and 2 because they are relatively more robust to possible omitted variable bias problems. Method 1 was preferred over method 2 because calculation of marginal effects and associated p-values, taking into account site-specific effects, was feasible using the “margins” command in Stata with that method. We emphasize, however, that the results on the coefficients that we obtained for all methods were very similar, so our conclusions are in fact robust to the choice of method.
8 For a clear exposition of these two perspectives, in the context of SSA's Project NetWork randomized trial, see Rupp, Bell, and McManus (1994).
9 See Ruiz-Quintanilla and others (2005/2006) for a study on enrollment of SSI recipients with psychiatric disabilities who were in an employment program that faced similar constraints on data and selection of explanatory variables.
10 The most likely explanation for missing letter dates was that the beneficiary had not been recruited because the relevant site had already reached its enrollment target and thus terminated recruitment.
11 The three variables in Table 1 relating to educational attainment (Edu_bach, Edu_hs, and Edu_nohs) were created using the census tract in which the beneficiary's residence address (from the MBR) was located, using the age/gender group within the block group or tract corresponding to the beneficiary. The age ranges we used for this purpose were 18–24, 25–34, 35–44, and 45–64.
12 Reported median earnings are for the census block group in which the beneficiary's residence address (from the MBR) is located. (In a minority of cases, only tract-level data were available.)
13 Variables defined in Table 1 with arithmetic transformations (for example, age and median earnings) are shown in Table 2 without such transformations.
14 Only 29,150 persons were included in Table 2 and in all our other analyses because some individuals had missing data. (Note that in the flowchart, although there are 29,745 potential plus possibly potential enrollees, 595 of those persons were dropped from our analyses because they did not have complete data for all variables used in the study. Thus, only 2.0 percent of these persons were dropped from the study—with this exclusion having virtually no impact on the results.)
15 Specific information on all other explanatory variables included in the exploratory regressions is available from the authors upon request.
16 Further detailed information on sensitivity tests and results for our initial exploratory regressions is available from the authors upon request.
17 Coefficients and corresponding standard error estimates for all regressions shown in Table 3 are available from the authors upon request.
18 In the New York WORKS project, randomization occurred before contacting the eligible beneficiaries; there was no final enrollment stage for the control group (Ruiz-Quintanilla and others 2005/2006).
19 In the case of TTW, while we refer to “enrollment” rates, note that all DI beneficiaries and SSI recipients received Tickets and had the opportunity to use them.
20 The TTW program began mailing Tickets to beneficiaries in the phase-1 states in early 2002, almost 3 years before December 2005. The fact that the TTW recruitment activities consisted of initial mail contacts with essentially no follow-up or substantial outreach efforts thereafter presumably contributed to a low enrollment rate (Stapleton and others 2008).
The New York WORKS study targeted only SSI recipients (of whom about 25.0 percent received concurrent SSI/DI benefits) with psychiatric disabilities who were residing in either Erie County or New York City. In its 30-month recruitment period, initial outreach was conducted by mail, and follow-up occurred only if a beneficiary returned the mailed response card with expression of interest (Ruiz-Quintanilla and others 2005/2006).
Project NetWork targeted SSI, DI, and concurrent beneficiaries with all types of disabilities who were residing in one of eight areas around the country. It used both mailings and in-person outreach efforts. Although there was a 15-month recruiting phase, sites that reached their enrollment targets discontinued enrollment in a shorter time period (Burstein, Roberts, and Wood 1999).
Both the New York WORKS project and Project NetWork differed from the MHTS by including persons older than age 55; in the New York WORKS project, those persons comprised more than 30.0 percent of the study subjects.
21 Note that a large majority of beneficiaries entering a TWP complete it and enter an EPE following the TWP completion (Stapleton and others 2008).
22 Differences between the findings of our study and those of others could also arise from differences in functional form. In particular, time on rolls and age (which may be correlated with time on rolls) were tested as continuous variables (including squared terms in exploratory regressions) in our models. The Project NetWork and New York WORKS studies treated those variables as categorical (that is, as step functions).
23 One should also bear in mind that the analyses reported in this Appendix combined both test and validation cases. Thus, a caveat to our findings is that all reported p-statistics in the accompanying Appendix table are potentially biased downward because of the large number of exploratory regressions on the test-sample data, as described in the Reduced-Form Enrollment Regression Results section.
References
Altonji, Joseph G., and Rebecca M. Blank. 1999. “Race and Gender in the Labor Market.” In Handbook of Labor Economics, Vol. 3C, chap. 48, edited by Orley C. Ashenfelter and David Card, 3143–3260 New York, NY: Elsevier.
Bond, Gary R., Robert E. Drake, and Deborah R. Becker. 2008. “An Update on Randomized Controlled Trials of Evidence-Based Supported Employment.” Psychiatric Rehabilitation Journal 31(4): 280–290.
Burns, Tom., Jocelyn Catty, Sarah White, Thomas Becker, Marsha Koletsi, Angelo Fioritti, Wulf Rössler, Toma Tomov, Jooske van Busschbach, Durk Wiersma, and Christoph Lauber—for the EQOLISE Group. 2009. “The Impact of Supported Employment and Working on Clinical and Social Functioning: Results of an International Study of Individual Placement and Support.” Schizophrenia Bulletin 35(5): 949–958.
Burstein, Nancy R., Cheryl A. Roberts, and Michelle L. Wood. 1999. Recruiting SSA's Disability Beneficiaries for Return-to-Work: Results of the Project NetWork Demonstration. Bethesda, MD: Abt Associates, Inc.
Cameron, A. Colin, and Pravin K. Trivedi. 2010. Microeconometrics Using Stata, Revised Edition. College Station, TX: Stata Press.
Campbell, Kikuko, Gary R. Bond, and Robert E. Drake. 2009. “Who Benefits from Supported Employment: A Meta-Analytic Study.” Schizophrenia Bulletin 37(2): 370–380.
Drake Robert E., William Frey, Gary R. Bond, Howard H. Goldman, David Salkever, Alexander Miller, Troy A. Moore, Jarnee Riley, Mustafa Karakus, and Roline Milfort. 2013. “Assisting Social Security Disability Insurance Beneficiaries with Schizophrenia, Bipolar Disorder, or Major Depression in Returning to Work.” American Journal of Psychiatry 170(12): 1433–1441.
Frey William D., Susan T. Azrin, Howard H. Goldman, Susan Kalasunas, David S. Salkever, Alexander L. Miller, Gary R. Bond, and Robert E. Drake. 2008. “The Mental Health Treatment Study.” Psychiatric Rehabilitation Journal 31(4): 306–312.
Greene, William. 2004. “The Behaviour of the Maximum Likelihood Estimator of Limited Dependent Variable Models in the Presence of Fixed Effects.” Econometrics Journal 7(1): 98–119.
Hall, Laura Lee, Abigail C. Graf, Michael J. Fitzpatrick, Tom Lane, and Richard C. Birkel. 2003. Shattered Lives: Results of a National Survey of NAMI Members Living with Mental Illness and Their Families. Treatment/Recovery Information and Advocacy Database (TRIAD) Report. Arlington, VA: National Alliance for the Mentally Ill (July).
Hilbe, Joseph M. 2009. Logistic Regression Models (Tests in Statistical Science). Boca Raton, FL: Chapman and Hall/CRC.
Kennedy, Peter. 2008. A Guide to Econometrics, 6th ed. Hoboken, NJ: Wiley-Blackwell.
Killingsworth, Mark R., and James J. Heckman. 1986. “Female Labor Supply: A Survey.” In Handbook of Labor Economics, Vol. 1, chap. 2, edited by Orley C. Ashenfelter and Richard Layard, 103–204. New York, NY: Elsevier.
Lehman, Anthony F., Donald M. Steinwachs, and the survey co-investigators of the PORT Project. 1998. “Patterns of Usual Care for Schizophrenia: Initial Results from the Schizophrenia Patient Outcomes Research Team Client Survey.” Schizophrenia Bulletin 24(1): 11–20.
Mashaw, Jerry L., and Virginia P. Reno, eds. 1996. Balancing Security and Opportunity: The Challenge of Disability Income Policy. Report of the Disability Policy Panel. Washington, DC: National Academy of Social Insurance.
Pencavel John. 1986. “Labor Supply of Men.” In Handbook of Labor Economics, Vol. 1, chap. 1, edited by Orley C. Ashenfelter and Richard Layard, 3–102. New York, NY: Elsevier.
Ruiz-Quintanilla, S. Antonio, Robert R. Weathers II, Valerie Melburg, Kimberly Campbell, and Nawaf Madi. 2005/2006. “Participation in Programs Designed to Improve Employment Outcomes for Persons with Psychiatric Disabilities: Evidence from the New York WORKS Demonstration Project.” Social Security Bulletin 66(2): 49–79.
Rupp, Kalman, Stephen H. Bell, and Leo McManus. 1994. “Design of the Project NetWork Return-to-Work Experiment for Persons with Disabilities.” Social Security Bulletin 57(2): 3–20.
[SSA] Social Security Administration. 2012. Annual Statistical Report on the Social Security Disability Insurance Program, 2011 (Table 24). Washington, DC: Office of Retirement and Disability Policy, Office of Research, Evaluation, and Statistics.
Stapleton, David, Gina Livermore, Craig Thornton, Bonnie O'Day, Robert Weathers, Krista Harrison, So O'Neil, Emily Sama Martin, David Wittenburg, and Debra Wright. 2008. Ticket to Work at the Crossroads: A Solid Foundation with an Uncertain Future. http://www.socialsecurity.gov/disabilityresearch/twe_reports/twe4.htm.
Studenmund, A. H. 2011. Using Econometrics, 6th ed. Boston, MA: Addison-Wesley.
Wang, Philip S., Patricia Berglund, and Ronald C. Kessler. 2000. “Recent Care of Common Mental Disorders in the United States: Prevalence and Conformance with Evidence-Based Recommendations.” Journal of General Internal Medicine 15(5): 284–292.
Westat. 2011. Mental Health Treatment Study: Final Report. http://www.socialsecurity.gov/disabilityresearch/documents/MHTS_Final_Report_508.pdf.
Wright, Benjamin D., and Graham A. Douglas. 1975. Better Procedures for Sample-Free Item Analysis. Research Memorandum No. 20. Chicago, IL: University of Chicago, Department of Education, Statistical Laboratory.