Accounting for Geographic Variation in Social Security Disability Program Participation
Social Security Bulletin, Vol. 78 No. 2, 2018 (released May 2018)
There is wide geographic variation in Social Security Disability Insurance and Supplementary Security Income participation across the United States. Some policymakers and members of the public may assume that interregional administrative inconsistencies are a major reason for the geographic variation. To test this assumption, and to reveal other potential explanations for the variation, we decompose the total variation into components by examining regional differences in disability prevalence and in program participation among persons with disabilities as well as the correlation between those two factors. We further decompose the variation in participation among persons with disabilities into socioeconomic components. Our findings strongly suggest that geographic variation in program participation is mainly an indication of geographic variation in disability prevalence and socioeconomic characteristics and that inconsistency in program administration is not a major reason for the variation.
The authors are with the Center for Health Policy and Research at the University of Massachusetts Medical School.
Acknowledgments: The authors thank David Stapleton; his thoughtful comments and suggestions on an earlier version greatly improved the article. We also thank Michael Leonesio and three anonymous reviewers for their very helpful comments and suggestions.
Note: The research reported herein was performed pursuant to a grant (no. DRC12000001) from the Social Security Administration and funded as part of the Disability Research Consortium. Contents of this publication are not copyrighted; any items may be reprinted, but citation of the Social Security Bulletin as the source is requested. The findings and conclusions presented in the Bulletin are those of the authors and do not necessarily represent the views of the Social Security Administration.
Introduction
ACS | American Community Survey |
CAPUMA | county-aligned Public Use Microdata Area |
DI | Disability Insurance |
PUMA | Public Use Microdata Area |
SSA | Social Security Administration |
SSI | Supplemental Security Income |
There is wide geographic variation in Social Security Disability Insurance (DI) and Supplementary Security Income (SSI) participation rates (participants as a percentage of the working-age population). For the period 2009–2011, we calculate DI participation rates ranging from 0.4 percent in Aleutians West County, Alaska to 21.0 percent in Buchanan County, Virginia. SSI participation rates range from 0.1 percent in Pitkin County, Colorado to 21.0 percent in Owsley County, Kentucky.1 The variation is large; however, what accounts for the variation is not well understood, and its significance for DI/SSI is not known.2
One potential explanation is inconsistent program administration. The Social Security Advisory Board (2001, 2012a, 2012b) has reported geographic inconsistencies in DI/SSI program administration—specifically, in allowance and denial rates, bases for initial awards and denials, and disability examiner salary levels and attrition rates—and these inconsistencies could underlie geographic variation in program participation. The Board has expressed concern that the Social Security Administration (SSA) lacks the information needed to determine whether the inconsistencies in program administration cause inconsistent outcomes—and this in turn raises questions about program integrity (Social Security Advisory Board 2001). Besides inconsistent program administration, other plausible explanations for geographic variation in DI/SSI participation include variations in disability prevalence, demographic characteristics, employment opportunities for people with disabilities, and health care and public assistance program access.
In the absence of empirical analysis explaining the geographic variation in program participation, some policymakers and members of the public assume that administrative inconsistency is a major underlying cause.3 In this study, we decompose the geographic variation in participation into its component variations. This approach allows us to determine the relative importance of each component. We find that nearly all of the variation in program participation is explained by variation in disability prevalence and certain socioeconomic factors. This finding strongly suggests that inconsistent program administration is not a major cause of the geographic variation.
Participation in DI/SSI is contingent on severe disability; conceptually, participants are thus restricted to the subpopulation of persons with disabilities and cannot be part of the subpopulation of persons without disabilities. For this reason, the geographic variation in DI/SSI participation is the composite of two component geographic variations: that of the prevalence of disability and that of DI/SSI participation among persons with disabilities. We therefore seek to determine how much of the overall variation is attributable to each of those two components. In addition, we further decompose the variation in DI/SSI participation among persons with disabilities into subcomponents defined by selected demographic and labor market characteristics.
To the best of our understanding, this research is the first to use variance decomposition methods to analyze the geographic variation. Prior research has generally used regression methods, which are well-suited to describing associations and to estimating the total explained variance. However, regression methods do not account for the relative importance of the components to the total variation. Also to the best of our understanding, our research is among the first to examine the geographic variation in participation specifically among persons with disabilities.4 Prior research examined the variation among all persons, with and without disabilities. As we discuss in the Methods section, the interpretation of results and assessment of policy implications are more straightforward when based on analysis of persons with disabilities.
This article consists of eight sections, including this introduction. The second section reviews the prior literature. The third section discusses our methods and describes the variance decomposition measures. The fourth section discusses our data. The fifth section presents our results. It includes statistical tables and thematic maps that describe the geographic variations in DI/SSI participation, disability prevalence, and DI/SSI participation among persons with disabilities. It also includes the variance decomposition estimates. The final three sections respectively discuss the findings, consider the limitations of the study, and summarize the implications of the analysis.
Previous Literature
Conceptually, participation in DI or SSI is determined by the interaction of the demand for DI/SSI among people with disabilities and the supply of benefits from the federal government (Rupp and Stapleton 1998). Demand is driven by the relative costs and benefits of individuals' participation versus nonparticipation. The supply of benefits is determined by program policy and operational procedures. Abundant literature examines factors that affect the demand and supply of benefits. Much of that research was conducted to explain the growth in the DI caseload, which has approximately doubled in the last 30 years (Daly, Lucking, and Schwabish 2013). A substantial majority of the growth can be attributed to changes in the size and age/sex composition of the labor force (Liebman 2015; Zayatz 2015; Pattison and Waldron 2013; Daly, Lucking, and Schwabish 2013); however, studies indicate that other factors also affect the supply of and demand for disability benefits. The literature informs our study by identifying factors affecting supply or demand that vary across geographic regions.
The geographic variation in DI/SSI participation was first described in the early 1990s in studies such as McCoy, Davis, and Hudson (1994) and Nelson (1994). Many subsequent studies have found that area differences in labor markets affect benefit demand (for example, McVicar 2006). Using local-area labor market variations based on a coal boom and subsequent bust, Black, Daniel, and Sanders (2002) found that permanent job creation and destruction have a larger effect on DI and SSI use than transitory local-area labor market changes do. A number of studies have found that adverse state conditions such as high unemployment or employment contractions are associated with increases in DI application (Autor and Duggan 2003; Burkhauser, Butler, and Weathers 2001/2002; Rupp and Stapleton 1995; Soss and Keiser 2006; Guo and Burton 2012; Coe and others 2011). In research indirectly related to demand, studies have found a negative association between state unemployment rates and disability program allowance rates, suggesting that areas with higher unemployment have a lower proportion of DI applicants with severe disabilities and that administrative procedures are likely to screen out applications filed by claimants without severe disabilities (Rupp 2012; Strand 2002; Rupp and Stapleton 1995).
State policies and programs may also affect the demand for DI. Studies have found that state variation in health insurance availability and cost, mandated employer-sponsored disability insurance, general assistance, unemployment benefits, and workers' compensation affect DI application rates (Maestas, Mullen, and Strand 2013; Coe and others 2011; Guo and Burton 2012; Rupp and Stapleton 1995; Rutledge 2012).
Studies have also investigated possible inconsistencies in program administration and whether state-by-state variations could affect DI/SSI supply. Strand (2002) estimated that approximately half of the interstate variation in allowance rates is associated with economic, demographic, and health factors external to program administration. Studies have suggested that allowance rates are affected by political or bureaucratic factors, as governors and other state officials exert influence on program administration (Iyengar and Mastrobuoni 2014; Keiser 2001). Woehl (2015) found that increasing workloads among disability determination workers and administrative law judges resulted in greater likelihood that the disability decision would err “on the side of awarding benefits.”
Using regression methods, some studies have decomposed the state variation in DI/SSI application or participation rates into “explained” and “unexplained” components. Rupp (2012) estimated that demographic factors, diagnostic factors, unemployment rates, state fixed effects, and time fixed effects accounted for approximately 75 percent of the state variation in DI and SSI allowance rates. Coe and others (2011) estimated that health status, demographics, and employment status explain over 70 percent of the state variation in DI application rates. Soss and Keiser (2006) estimated that differences in disability prevalence, demographic factors, unemployment, poverty, the availability of civil society organizations, the political ideology of state officials, and the generosity of state public assistance explain 59 percent of state variation in DI application volume and 75 percent of state variation in SSI application. Ruffing (2015) estimated that differences in socioeconomic characteristics such as education, median age, foreign-born share of the population, industry mix, poverty rate, and unemployment rate account for 84 percent of state variation in persons participating in either DI or SSI. The fact that these studies estimate comparable percentages of explained variation using different explanatory variables suggests high correlations between observed and unobserved explanatory factors. It is therefore difficult to determine the relative importance of discrete components in accounting for the overall variation. As we discuss below, the variance decomposition methods used in this study better account for the contributions of the discrete components.
Geographic variation in disability prevalence and health condition may also affect DI and SSI demand. Some studies control for disability or health when estimating the effects of supply or demand factors. Other research has estimated state-level variation in health or disability effects directly. Rutledge and Wu (2014) found that poor health increases SSI application and participation. Similarly, Coe and others (2011) found that poor health increases DI application. Soss and Keiser (2006) found that DI and SSI application rates are positively associated with disability prevalence. These studies include health or disability as an independent factor in regression models of DI/SSI participation. Below, we discuss the advantages of separately analyzing the variations in disability prevalence and in program participation among the population with disabilities.
Methods
We decompose the variation in DI/SSI participation in three operations. We first decompose the overall variation in participation into two component variations: in disability prevalence and in the participation rate among persons with disabilities. Second, we decompose the variation in program participation among persons with disabilities into socioeconomic subcomponents. We do this by using principal-components analysis to determine uncorrelated components, for which the variance contribution of each subcomponent can then be calculated. Finally, we decompose the unexplained variation from the second decomposition into two factors, within-state and between-state variation, using fixed-effect regression methods.
The variance decomposition methods apply equally to the DI and SSI programs. However, because DI is a social insurance program and SSI is a means-tested program, we expect that the variance decomposition accounting for them will differ and we produce separate variance decomposition estimates for each. Nevertheless, recall that we use the term “DI/SSI participation” in this article to refer to participation in either program. Thus, to reiterate, it may include but is not restricted to concurrent participation in both programs.
Disability Prevalence and Program Participation Decomposition
We define the variable g as the geographic area index for each applicable measure of geographic variation. The DI/SSI participation rate (part) in a geographic area is defined by equation 1:
where npart is the number of DI/SSI participants and ntotal is the total number of working-age persons.
The DI/SSI participation rate among persons with disabilities (partdis) is defined by equation 2:
where ndisability is the number of working-age persons with disabilities.
Disability prevalence (disprev) is defined by equation 3:
By definition, partg is equal to the product of disprevg and partdisg (equation 4):
The variance relationship is given in equation 5:
which indicates that the variance of part is the variance of the product of disprev and partdis. The variance of part is dependent on the variance of disprev, the variance of partdis, and the correlation between disprev and partdis. Our objective is to determine the relative contributions of disprev and partdis to the variance of part. To facilitate that determination, we use the natural log transformation of equation 4, which is additive (equation 6):
The variance relationship is given in equation (7):
We define three variance decomposition measures. The first is the percentage of variance in the natural log of the DI/SSI participation rate attributed to the natural log of disability prevalence; we call this measure percentage variance in disability or PVdisability (equation 8):
The second is the percentage of variance in the natural log of DI/SSI participation among persons with disabilities; we call this measure percentage variance in participation or PVparticipation (equation 9):
The third is the percentage of variance in the natural log of DI/SSI participation among persons with disabilities that is due to the correlation between the prevalence of disability and the DI/SSI participation rate; this measure, the percentage variance in correlation, or PVcorrelation, accounts—as its name suggests—for the variation that is due to correlation (equation 10):
If the prevalence of disability disprev and the DI/SSI participation rate among those with disabilities partdis are not correlated, the sum of PVdisability and PVparticipation is approximately 100 percent. If disprev and partdis are positively correlated, the sum of PVdisability and PVparticipation is less than 100 percent and the sum decreases as the positive correlation increases. Conversely, the sum is greater than 100 percent in the case of a negative correlation and the sum increases as the negative correlation increases.
Participation Among Persons with Disabilities Decomposition
To reiterate, PVparticipation and PVdisability indicate how much of the variance in DI/SSI participation is attributed to variation in DI/SSI participation among persons with disabilities and how much is attributed to variation in disability prevalence. We use principal-components analysis to further decompose the variation in DI/SSI participation among persons with disabilities into socioeconomic subcomponents. This study does not further decompose the variation in disability prevalence, which we defer to future research.
As noted earlier, area-level patterns in numerous socioeconomic characteristics are expected to be associated with DI/SSI participation among persons with disabilities. These include the relative prevalence of certain demographic, income, and poverty characteristics; disability types; labor market conditions; and public assistance participation and health insurance coverage rates. We expect that some area-level socioeconomic characteristics will be endogenous if they are based on the population of persons with disabilities. For example, an area may have a low employment rate among persons with disabilities because of both the negative employment effects of DI/SSI participation and the area's unfavorable economic conditions. When we expect that a characteristic would be endogenous if it were calculated based on the population of persons with disabilities, we address that limitation by calculating variables based on the population of persons without disabilities. We do so because we expect that the area-level conditions reflected in characteristics based on persons without disabilities are also experienced by persons with disabilities. For example, a high labor-force participation rate likely indicates the area labor market conditions experienced by persons both with and without disabilities. In other words, we define variables based on the population of persons with disabilities when the characteristic (for example, percentage female) is not expected to be affected by DI/SSI participation. Box 1 shows which population subgroup (with or without disabilities) we used to calculate each variable. An ordinary least squares regression of DI/SSI participation among persons with disabilities versus socioeconomic characteristics would provide an indication of overall explained variation; however, because the variables are correlated, it would not support estimates of the variance contribution of separate subcomponents (for example, labor market conditions). To estimate the subcomponent contributions, we use principal-component analysis.
Population with disabilities |
---|
Demographics |
Average age |
Percentage— |
Female |
Black |
Hispanic |
Not speaking English at home |
Born in United States |
U.S. citizen |
Never married |
High school diploma or less |
Disability |
Percentage reporting difficulty with— |
Hearing |
Independent living |
Self-care |
Vision |
Percentage reporting— |
Ambulatory difficulty |
Cognitive difficulty |
Population without disabilities |
Income and poverty |
Percentage in poverty a |
Average dollar amount of annual— |
Personal income |
Household income |
Earned income |
Percentage with— |
Public assistance |
Health insurance coverage |
Labor market indicators |
Labor force participation rate among— |
Men |
Women |
Self-employment rate |
Average hours usually worked per week |
Percentage of workers with 26 or fewer weeks worked |
Occupation type |
Service |
Production |
Sales |
Construction and maintenance |
Management |
Industry group |
Manufacturing |
Education and health services |
Professional and business services |
Wholesale and retail trade |
Leisure and hospitality |
Other industries |
SOURCE: Authors' definitions. |
a. Having income of less than 100 percent of the federal poverty level. |
By using principal-component analysis, we transform the socioeconomic characteristics into a smaller set of subcomponents that are uncorrelated. The variance contribution of each subcomponent to the total variance in partdisg can be determined by the square of the correlation between partdisg and the subcomponent. Because the subcomponents are a transformation of the original socioeconomic variables, a given subcomponent's meaning is not obvious. To determine its meaning, we examine the correlation of a given subcomponent with the original variables. For example: Referring to the variables in Box 1, a subcomponent that had a strong positive correlation with area average annual personal income, average annual household income, and average annual earned income and had a strong negative correlation with area poverty level was interpreted to represent area income and poverty. We will revisit the subcomponents and discuss their correlations in the Results section under “Participation Among Persons with Disabilities Decomposition Estimates.”
Unexplained Between-State and Within-State Decomposition
Principal-component analysis decomposes the variance in DI/SSI participation among persons with disabilities into observed socioeconomic subcomponents. However, the principal components do not capture all of the variance of the original socioeconomic variables; unobserved factors also account for some of the variance. Further, the principal-component analysis was conducted using substate geographic areas and it is possible that some of the unaccounted variance may occur at the state level. For example, differing state welfare policies may result in state-level variation in DI/SSI participation among persons with disabilities. It is also possible that factors that vary between states but are not determined by state policy—for example, employment discrimination against persons with disabilities, as well as attitudes about employment among persons with disabilities—may contribute to state-level variation in DI/SSI participation. To determine the state-level variation, we first estimated a regression without state fixed effects (total unexplained) and then added state fixed effects to determine how much more of the variation those variables explained (unexplained between-state).
Advantages of Variance Decomposition Methods
The variance decomposition approach has advantages over the regression approach used in prior research such as Rupp (2012), Coe and others (2011), Soss and Keiser (2006), and Ruffing (2015). For illustrative purposes, we assume that the data-generating processes for disprev and partdis are represented by equations 11 and 12. We assume that these two processes differ. For example, we expect that age has an independent effect on both disprev and partdis:
In equation 11, Xg is a vector of variables affecting disprev and β is a vector of their effects. In equation 12, Yg is a vector of variables affecting partdis and α is a vector of their effects. Some variables may independently affect both disprev and partdis and some variables may separately affect only disprev or partdis. For variables that affect both data-generating processes, the magnitudes and signs of the effects may differ. Using a research method that examines the variation in part directly, for example by using regression methods to estimate the parameters of equation 13,
will reveal associations and the total explained variation; however, the associations will not reveal the effects or explained variance of the separate data-generating processes (equations 11 and 12).
The estimates of equation 13 will not determine how much of the explained variation is because of disprev or partdis or the correlation between disprev and partdis. It will be difficult to interpret the estimated effects, for example the effect of age, because the estimate will not reveal the independent effects on disprev or partdis. Thus, studies based on models similar to equation 13 limit researchers' ability to explain the variation in DI/SSI participation, interpret the results, and assess policy implications. Separate analysis of the variation in disprev or partdis is preferred.
Data
We use data from the 2009–2011 American Community Survey (ACS) Public Use Microdata Sample to estimate the following substate statistics: the number of persons with disabilities, the number of working-age (18–64) persons, and socioeconomic characteristics. We choose the years 2009–2011 because substate geographic boundaries and ACS disability questions were consistent during that period. We do not include individuals living in institutional group quarters because DI/SSI participation is precluded for a large majority of the group-quarters population—those who are incarcerated—and the data do not allow us to differentiate that population from those living in other institutional group quarters, such as nursing homes.
We first determine the number of persons with disabilities. An ACS respondent who answers “yes” to any of the following questions is considered to have a disability:
- Is this person deaf or does he/she have serious difficulty hearing?
- Is this person blind or does he/she have serious difficulty seeing even when wearing glasses?
- Because of a physical, mental, or emotional condition, does this person have serious difficulty concentrating, remembering, or making decisions?
- Does this person have serious difficulty walking or climbing stairs?
- Does this person have difficulty dressing or bathing?
- Because of a physical, mental, or emotional condition, does this person have difficulty doing errands alone such as visiting a doctor's office or shopping?
We use publicly available data from SSA reports to determine the county-level numbers of DI and SSI participants (SSA 2012b, Table 4; 2012c, Table 2). For DI participants, we observe disabled workers but do not include disabled widow(er)s or disabled adult children because data for those groups were not available across geographic areas. In 2011, there were approximately 8.5 million disabled workers and 1.2 million disabled widow(er)s and disabled adult children (SSA 2015, Table 3). SSI payments include both federal and state supplementation payments. The available data do not distinguish federal SSI recipients from recipients of federally administered state supplementation payments across geographic areas. In December 2010, there were approximately 6.5 million federal payment recipients and 167,000 state supplementation–only recipients (SSA 2012a, Table 7.A1).
Our analysis assumes that people who meet the SSA standard for disability (with the exception of the requirement that the person's earnings are less than the amount that signifies substantial gainful activity) are a subgroup of the population of persons with disabilities.5 However, if ACS survey data are used to identify persons with disabilities, that assumption is not necessarily valid. Using Current Population Survey (CPS) data matched with SSA administrative records, Burkhauser, Houtenville, and Tennant (2014) found that the CPS questions on disability only identified approximately 63 percent of DI and SSI beneficiaries. The CPS and the ACS ask the same disability questions, suggesting that disability is also underreported in the ACS. The underreporting of disability will not substantially bias the decomposition estimates, provided the underreporting is not associated with DI/SSI participation.6
However, it is possible that the underreporting of disability is associated with DI/SSI participation. For example, DI/SSI participants, wishing to justify their participation, may be more likely to report their disabilities than are nonparticipants with equivalent disabilities. This justification bias would result in a positive correlation between the bias in the estimates of disability prevalence (disprev) and of DI/SSI participation (part), which would in turn bias the variance decomposition estimates.7 In such a case, the variance contribution of disability prevalence (PVdisability ) would be overestimated and the variance contribution of DI/SSI participation among persons with disabilities (PVparticipation ) would be underestimated. The bias in the variance contribution of correlation (PVcorrelation ) depends on the relative dispersion of disability prevalence compared with that of DI/SSI participation among persons with disabilities. The coefficient of variation (CV) is a measure of the standardized dispersion of a distribution and is defined as the ratio of the standard deviation to the mean. If the CV of disability prevalence is greater than the CV of DI/SSI participation among persons with disabilities, the variance contribution of correlation will be underestimated. If the opposite is true, the variance contribution of correlation will be overestimated.8 We assess the potential effect of justification bias on the variance decomposition estimates in the Results section under “Bias Assessment.”
Geographic Regions
ACS data on disability prevalence are available at the Public Use Microdata Area (PUMA) level of geographic detail. PUMAs are defined by the Census Bureau and consist of intrastate regions of approximately 100,000 to 200,000 people. SSA data on DI/SSI participation are available at the county level for all but 104 counties.9 To facilitate analysis of disability prevalence and DI/SSI participation, we define a common geography that combines PUMAs and counties and call the resulting units county-aligned Public Use Microdata Areas (CAPUMAs). We define CAPUMAs as the smallest areas that intersect one or more PUMAs and one or more counties. For example, for areas where the PUMA boundary matches the county boundary, the CAPUMA is equivalent to both the PUMA and the county. For areas where the PUMA contains multiple complete counties, the CAPUMA is equivalent to the PUMA. Conversely, for areas where the county contains multiple complete PUMAs, the CAPUMA is equivalent to the county.10 We combined the 2,069 PUMAs and 3,142 counties into 937 CAPUMAs with estimated working-age (18–64) populations ranging widely, from about 50,000 to about 6.3 million people.
Results
DI participation varies widely across CAPUMAs. Overall, the average DI participation rate among working-age persons is 5.4 percent, with a standard deviation of 2.1 (Table 1), and the CAPUMA-level participation rates range from 1.0 percent to 16.6 percent, as indicated by the lower- and upper-bound values for the participation-rate quintiles shown in the legend to Chart 1.
Statistic | Disability prevalence | DI participation rate | SSI participation rate | ||
---|---|---|---|---|---|
Persons with disabilities | Overall | Persons with disabilities | Overall | ||
Mean | 12.0 | 45.1 | 5.4 | 22.7 | 2.8 |
Standard deviation | 3.9 | 7.9 | 2.1 | 7.9 | 1.6 |
75th percentile | 14.2 | 50.2 | 6.5 | 26.3 | 3.5 |
Median | 11.4 | 45.1 | 5.1 | 21.2 | 2.5 |
25th percentile | 9.1 | 39.8 | 1.7 | 17.4 | 1.8 |
SOURCE: Authors' calculations based on ACS data and SSA reports. |
Geographic distribution of DI participation: CAPUMAs, by working-age participation-rate quintile. 2009–2011
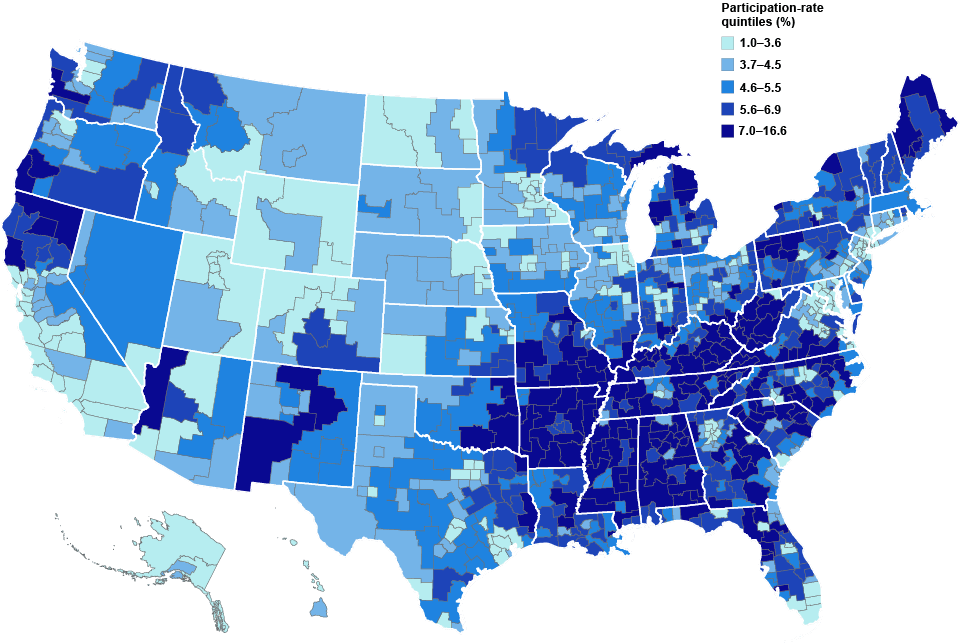
Chart 1 is a choropleth map showing the variation in DI participation across the United States by CAPUMA. Because of varying population density across areas, some geographically large CAPUMAs represent very few people, and some small areas represent many. For example, the Coconino County, Arizona CAPUMA land area is 18,600 square miles, yet it includes only about 90,000 working-age people and approximately 2,000 DI beneficiaries. By contrast, the Kings County (Brooklyn), New York CAPUMA is small, approximately 71 square miles, yet it includes 1.6 million working-age people and about 51,000 DI beneficiaries.
Chart 1 shows both within-state and between-state variation. In Georgia, for example, DI participation is relatively low in the vicinity of Atlanta and higher in other areas of the state. The two CAPUMAs that include greater Atlanta, Fulton County and Dekalb County, have respective DI participation rates of 2.9 percent and 3.1 percent. By contrast, the DI participation rate is 7.9 percent in the southeast Georgia CAPUMA containing Atkinson, Bacon, Brantley, Charlton, Cinch, Coffee, Pierce, and Ware Counties.
Chart 1 indicates that many high-population urban areas have relatively low DI participation. The CAPUMAs in the northeast corridor from Washington, DC to New York City and those that include and surround Atlanta, Chicago, San Antonio, Dallas, Los Angeles, and San Francisco are generally in the lowest quintile for DI participation. For example, the CAPUMA of Cook County, Illinois, which includes Chicago, has a working-age population of 3.3 million and a DI participation rate of 3.2 percent. Similarly, the Harris County, Texas CAPUMA includes Houston and has a working-age population of 2.6 million and a DI participation rate of 2.6 percent. Participation is generally low in CAPUMAs in the midwestern and western states of North Dakota, South Dakota, Nebraska, Montana, Wyoming, and Utah. Chart 1 also indicates high DI participation in CAPUMAs across southeastern states including Arkansas, Mississippi, Alabama, Tennessee, Kentucky, and West Virginia. For example, two adjacent CAPUMAs—one in eastern Kentucky (Boyd, Carter, Elliot, and Lawrence Counties) and one in western West Virginia (Lincoln, Logan, Mingo, and Wayne Counties)—have DI participation rates of 11.1 percent and 12.9 percent, respectively.
Chart 2 shows the same data for SSI participation that Chart 1 shows for DI. The national average SSI participation rate of 2.8 percent (standard deviation = 1.6) is lower than that for DI (Table 1); however, the CAPUMA-level range of SSI participation rates, from 0.2 percent to 13.7 percent (indicated in the legend to Chart 2), is roughly similar to that for DI. SSI participation varies both within and between states, as does DI participation. Also similar to DI is that many CAPUMAs in the northeast corridor, and in or surrounding other major U.S. cities, have relatively low SSI participation. However, there are exceptions; for instance, the Bronx County, New York CAPUMA and the city of Baltimore, Maryland CAPUMA have high SSI participation (6.6 percent and 6.2 percent, respectively). Chart 2 shows many high SSI participation CAPUMAs in southeastern states including Arkansas, Louisiana, Mississippi, Alabama, Georgia, Kentucky, and West Virginia. The four CAPUMAs with the highest SSI participation, all exceeding 10 percent, are in southeastern Kentucky.11
Geographic distribution of SSI participation: CAPUMAs, by working-age participation-rate quintile. 2009–2011
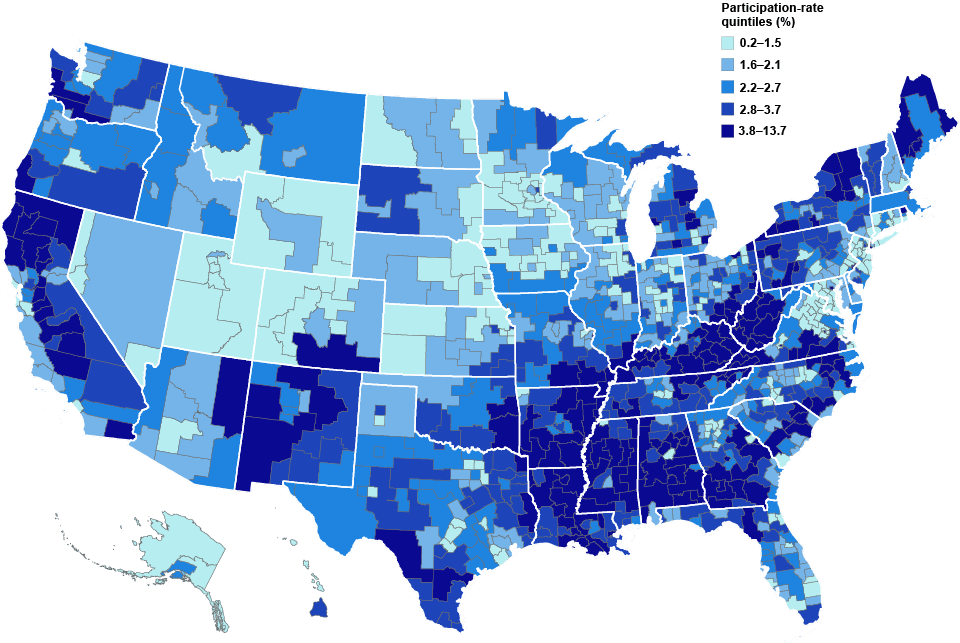
There is also wide geographic variation in disability prevalence and DI/SSI participation among persons with disabilities. Table 1 provides summary nationwide statistics. The average disability prevalence is 12.0 percent (standard deviation = 3.9). The average DI participation rate among working-age persons with disabilities is 45.1 percent (standard deviation = 7.9) and the average SSI participation rate among working-age persons with disabilities is 22.7 percent (standard deviation = 7.9).
Disability Prevalence and Program Participation Decomposition Estimates
To determine how much of the variance in DI/SSI participation across CAPUMAs is because of the variation in disability prevalence and how much is because of variation in DI/SSI participation among persons with disabilities, we estimated the variance decomposition measures PVdisability and PVparticipation, as described in the Methods section.
Approximately two-thirds (67.9 percent) of the variance in DI participation is attributable to the variation in disability prevalence and approximately one-fifth (21.2 percent) is attributable to the variation in DI participation among persons with disabilities (Table 2). The correlation between area disability prevalence and area DI participation among persons with disabilities is relatively low (0.12; not shown) and it accounts for approximately one-tenth (10.9 percent) of the variation in DI participation.
Statistic | DI | SSI |
---|---|---|
Disability prevalence | 67.9 | 37.5 |
Participation among persons with disabilities | 21.2 | 36.7 |
Correlation | 10.9 | 25.8 |
SOURCE: Authors' calculations based on ACS data and SSA reports. |
For SSI participation, the variance attributable to variation in disability prevalence (37.5 percent) and the variance attributable to variation in SSI participation among persons with disabilities (36.7 percent) are approximately equal. There is weak correlation between area disability prevalence and area SSI participation among persons with disabilities (0.31; not shown) and the correlation accounts for 25.8 percent of the variation.
The results provide an indication of the hypothetical variation in DI/SSI participation that would exist if there were variation in only one component, either disability prevalence or DI/SSI participation among persons with disabilities. For example, if disability prevalence were geographically constant, the cross-CAPUMA variation in DI participation would be approximately 21 percent of the current variation and the cross-CAPUMA variation in SSI participation would be approximately 37 percent of the current variation. Correspondingly, if program participation among persons with disabilities were geographically constant, the cross-CAPUMA variation in DI participation would be approximately 68 percent of the current variation and the cross-CAPUMA variation in SSI participation would be approximately 38 percent of the current variation.
Participation Among Persons with Disabilities Decomposition Estimates
To further decompose the variance of DI/SSI participation among persons with disabilities, we used principal-components analysis to define uncorrelated socioeconomic subcomponents. That analysis reduced the 37 socioeconomic variables shown in Box 1 to 12 subcomponents in 4 broad categories (Table 3). Those 12 subcomponents account for 84.1 percent of the total variation in the original variables. For each subcomponent, Table 3 also shows the variables that have strong or moderate correlations (positive or negative coefficients with an absolute value of at least 0.50). The subcomponent names were chosen to represent the general meanings of the correlated variables.12
Subcomponent | Percentage variance in participation attributable to the subcomponent | Correlated variables (with correlation coefficients) | ||||||||||||||
---|---|---|---|---|---|---|---|---|---|---|---|---|---|---|---|---|
DI | SSI | Positive correlations | Negative correlations | |||||||||||||
Demographics and disability | ||||||||||||||||
Hispanic/non-English | 10.4 | 1.6 |
|
|
||||||||||||
Black/low self-employment | 0.5 | 18.3 |
|
|
||||||||||||
Age/few cognitive difficulties | 6.1 | 0.1 |
|
|
||||||||||||
Female | 0.1 | 0.2 |
|
|
||||||||||||
Personal assistance needs | 7.4 | 5.7 |
|
|
||||||||||||
Income and poverty | ||||||||||||||||
Income | 1.0 | 8.1 |
|
|
||||||||||||
Labor market | ||||||||||||||||
Manufacturing | 3.6 | 0.1 |
|
|
||||||||||||
Labor force participation | 0.6 | 3.7 |
|
|
||||||||||||
Construction | 3.1 | 0.9 |
|
|
||||||||||||
Sales | 0.5 | 0.1 |
|
|
||||||||||||
Education/health services | 7.0 | 4.2 |
|
|
||||||||||||
Public assistance | ||||||||||||||||
Program participation | 2.4 | 10.3 |
|
|
||||||||||||
SOURCE: Authors' calculations based on ACS data and SSA reports. | ||||||||||||||||
NOTE: . . . = not applicable. |
Because the subcomponents are uncorrelated, we can calculate the percentage of the total variance in DI/SSI participation among persons with disabilities that is attributable to each subcomponent. For DI, the largest contributions to the variance are those of the Hispanic/non-English subcomponent (10.4 percent), the personal assistance needs subcomponent (7.4 percent), the education/health services subcomponent (7.0 percent), and the age/few cognitive difficulties subcomponent (6.1 percent). The Hispanic/non-English subcomponent is associated with lower levels of DI participation and the personal assistance needs, education/health services, and age/few cognitive difficulties subcomponents are associated with higher levels of DI participation (not shown).13
For SSI, the largest contributions to the area variance are those of the black/low self-employment subcomponent (18.3 percent), the public assistance program participation subcomponent (10.3 percent), and the income subcomponent (8.1 percent). The black/low self-employment subcomponent and the public assistance participation subcomponent are associated with higher levels of SSI participation and the income subcomponent is associated with lower levels of SSI participation (not shown). Taken together, these subcomponents likely indicate the economic conditions in an area. Areas with high levels of the black/low self-employment and public assistance subcomponents and low levels of the income subcomponent are likely to be economically disadvantaged.
Unexplained Between-State and Within-State Decomposition Estimates
Although the principal-components analysis decomposes the variance in DI/SSI participation among persons with disabilities into observed socioeconomic subcomponents, unobserved CAPUMA-level and state-level factors account for further variance. Regression analysis decomposes the additional variance into two factors: variance of unobserved CAPUMA-level factors and variance of unobserved state-level factors.
Ordinary least squares regression estimates of equation 12 indicate that CAPUMA-level factors accounted for 50 percent of the variation in DI participation among persons with disabilities and for 63 percent of the variation for SSI. Estimates of the fixed-effects model indicate that in combination, variation in observed CAPUMA-level characteristics and variation between states account for 66 percent of the variation in DI participation (an increase of 16 percentage points) and for 80 percent of the variation in SSI participation (an increase of 17 percentage points). As described in the Methods section, the increase may be attributed to unobserved variation in state policy (involving matters such as health insurance regulation or access to welfare programs) or in other state-level factors (such as employment discrimination against persons with disabilities or attitudes about employment among persons with disabilities). We are not able to determine how much of the increase is attributed to state policy and how much is attributed to other factors. As an approximation, the increase represents the upper limit of the variance attributable to unobserved state policy.14
Variance Decomposition Summary
We combine the CAPUMA-level disability/participation variance decomposition, the principal-components variance decomposition, and the regression analysis to obtain an overall decomposition summary (Table 4). For the principal-components variance decomposition, we use five subcomponent categories: demographics and disability, income and poverty, labor market, public assistance, and other.
Statistic | DI | SSI |
---|---|---|
Disability prevalence | 67.9 | 37.5 |
Participation among persons with disabilities | 21.2 | 36.7 |
Observed CAPUMA-level characteristics | ||
Demographics and disability | 5.2 | 9.5 |
Income and poverty | 0.2 | 3.0 |
Labor market | 3.1 | 3.3 |
Public assistance | 0.5 | 3.8 |
Other | 1.6 | 3.6 |
Unobserved characteristics | ||
CAPUMA-level | 3.4 | 6.2 |
State-level | 7.2 | 7.3 |
Correlation | 10.9 | 25.8 |
SOURCE: Authors' calculations based on ACS data and SSA reports. |
For DI, the variation in disability prevalence accounts for 67.9 percent of the geographic variation in total participation among working-age persons, variation in DI participation among persons with disabilities accounts for 21.2 percent, and correlation accounts for 10.9 percent. The contribution of participation among persons with disabilities is further decomposed into its components; 10.6 percentage points are attributed to variation in the observed CAPUMA-level characteristics combined, 3.4 percentage points are attributed to variation in unobserved CAPUMA-level characteristics, and 7.2 percentage points are attributed to unobserved state-level characteristics.
For SSI, the variations in disability prevalence and in SSI participation among persons with disabilities contribute approximately equally to the total variance (37.5 percent and 36.7 percent, respectively). Because disability prevalence and SSI participation among persons with disabilities are weakly correlated, correlation contributes approximately 25.8 percent to the total variance. The contribution of participation among persons with disabilities is further decomposed: 23.2 percentage points are attributed to variation in the observed CAPUMA-level characteristics combined, 6.2 percentage points are attributed to variation in unobserved CAPUMA-level characteristics, and 7.3 percentage points are attributed to variation in unobserved state-level characteristics.
Bias Assessment
As discussed earlier, the variance decomposition estimates may be biased if self-reports of disability are associated with DI/SSI participation. Justification bias is the most likely reason for an association between self-reports of disability and DI/SSI participation. If justification bias exists, some of the geographic variation in estimated disability prevalence would be attributed to that bias and some would be attributed to the true variation. To assess the possible magnitude of such bias, we estimate the correlation between area disability prevalence and area factors that we expect to be correlated with disability prevalence but are less vulnerable to justification bias. These include mortality, the proportion of persons with diabetes, the proportion of persons with fair or poor health, and the proportion of smokers. If justification bias accounts for a substantial portion of the variation in disability prevalence, we would expect a weak correlation. However, we find strong correlations between disability prevalence and the area factors, ranging from 0.8 to 0.9. This suggests that justification bias, if present, is small. It also suggests that any biases in the variance decomposition estimates associated with justification bias would be small.15
Discussion
There has long been concern about possible inconsistencies in DI and SSI program administration across geographic areas. Some observers might assume that inconsistencies in program administration are a major reason for the wide geographic variation in program participation. Our results strongly suggest otherwise. We find that nearly all of the geographic variation in program participation is attributable to variation in disability prevalence and socioeconomic factors and that very little of it could be associated with inconsistencies in program administration.
If geographic inconsistencies in program administration exist, then some people with disabilities may have an incentive to migrate. For example, areas with lenient DI/SSI approval processes might attract migration from areas with more restrictive processes, resulting in geographic variation in program participation. The decomposition results do not indicate such an occurrence. The analysis uses an ACS-based definition of disability that does not distinguish between DI/SSI participants and nonparticipants. If migration based on administrative inconsistency exists, we expect that it affects disability prevalence and DI/SSI participation among people with disabilities similarly. People migrating to a given area to improve their chances of receiving DI/SSI benefits would increase both that area's disability prevalence and its DI/SSI participation among persons with disabilities. If the migration were substantial, the correlation between disability prevalence and DI/SSI participation among persons with disabilities would be high. However, because our results indicate that the correlation is weak, such migration is not implied by the geographic variation in disability prevalence.16
Geographic inconsistencies in program administration could conceivably be reflected in variation in socioeconomic characteristics as well. For example, areas with poor economic conditions might be expected to have a higher demand for benefits and more lenient approval processes. In such cases, variation that is due to inconsistencies in program administration would be correlated with one or more principal subcomponents such as area income or poverty. Even if the correlations exist, the decomposition results indicate that inconsistent program administration would not be a major reason for the variation in overall DI/SSI participation. The contribution of each subcomponent of variation is small relative to the total variation. Also, the subcomponents are uncorrelated with one another and thus we do not expect that inconsistencies in program administration would be correlated with more than one subcomponent.
We find that geographic variation in disability prevalence is a major reason for the wide variation in DI/SSI participation. If there were no variation in disability prevalence across CAPUMAs, the variation in DI participation would be reduced by approximately 80 percent and the variation in SSI participation would be reduced by approximately 63 percent. What accounts for the wide geographic variation in disability prevalence across CAPUMAs is not well understood. Future research is needed. The correlations between disability prevalence and DI/SSI participation among persons with disabilities are weak, suggesting that the factors associated with the former differ from the factors associated with the latter. The variation in disability prevalence may exist because of variation in the incidence of disability or in net migration of persons with disabilities relative to persons without disabilities across geographic areas. The incidence of disability could vary across areas because of geographic variation in demographics, health care access or quality, health or disability risk behaviors, risk of injury, or disease prevalence. Net migration could vary because of labor market, cost-of-living, or other area differences. For instance, if individuals without disabilities are more likely than those with disabilities to migrate from areas that are economically weak to areas that are strong, the prevalence of persons with disabilities would increase in economically weak areas relative to that of strong areas. There are strong regional differences in disability prevalence. Analysis of these patterns might help to identify the reasons for the variation.
There are differences between the decomposition of SSI participation among persons with disabilities and that for DI. SSI is a means-tested program and, for the most part, only individuals living in very low-income households are eligible. Thus, we expect that area variation in SSI participation would be associated with variation in area economic conditions, resulting in higher participation in economically disadvantaged areas. Our findings support that expectation. We find that variations in area socioeconomic characteristics associated with economic disadvantage account for the largest contributions to variance in SSI participation among persons with disabilities.
In contrast with SSI, little of the variance in DI participation is associated with area characteristics indicating economic disadvantage. Instead, certain demographic factors contribute most to the DI variance. Areas with higher proportions of people who are Hispanic, speak a language other than English in the home, were not born in the United States, or are noncitizens have lower DI participation. This could reflect access limitations, ineligibility (not enough quarters of coverage), language barriers, discrimination, or other possible factors. It is also possible that the demand for DI varies by ethnicity or country of origin.17 Further research is needed to determine the causes.
Decomposition analysis also provides insight into how much unobserved state policy factors may contribute to geographic variation in DI/SSI participation. Although we find that unobserved state policies may contribute, their effect appears to be relatively small. Our fixed-effects estimates suggest that the upper limit of the contribution of unobserved state policies is approximately 7 percent.
The decomposition reveals the sources of approximately 90 percent of the variation in DI/SSI participation that Charts 1 and 2 illustrate. Why does some of the variation remain unexplained? There are a number of possible reasons. For example: Our methods rely on cross-sectional data that provide a current snapshot of area characteristics. However, DI/SSI participation depends on both current and past characteristics, such as long-term labor market trends. We are unable to account for characteristics in prior time periods that are uncorrelated with current characteristics. We are also not able to account for migration. DI/SSI participation may be affected by characteristics of a migrant's prior area of residence, for which we are not able to account. Also, area characteristics that we were unable to observe (such as employment discrimination and population density) may vary in ways that affect DI/SSI participation. Lastly, part of the unexplained variation is likely due to estimation errors that affect the survey data with which disability prevalence and area characteristics are calculated.
Publicly available ACS and SSA data for substate areas made this analysis possible. We merged ACS PUMA-level statistics with SSA county-level data to generate CAPUMA-level data. One shortcoming of this approach is that some of the CAPUMAs represent large populations, generally because some counties have large populations. Merging PUMAs and counties obscures some of the local-area variations, particularly in urban areas with dense populations. A PUMA-level analysis could offer improved results because it would more than double the number of observations, reveal urban-area variations, and provide study areas with consistent population sizes. Currently, however, DI/SSI participant counts are not available at the PUMA level. In addition, comparing subgroups that vary in DI/SSI participation rates, such as by age and sex, would improve the analysis. At present, substate DI/SSI participation counts by age and sex are not publicly available.
This study decomposes geographic variation in DI/SSI participation; however, the findings have implications beyond accounting for area variation. We discuss three. First, the decomposition analysis suggests that changes in disability prevalence, if they occur over time, will be reflected in DI participation changes. Disability prevalence is the predominant source of geographic variation in DI participation even though area labor markets and economic conditions can also vary widely. Changes in disability prevalence over time and in DI participation would likely have similar associations, suggesting that future changes in disability prevalence will proportionally change DI participation. Disability prevalence varies widely across areas, and accounting for this variation may provide insight into long-term trends in disability.
Second, the analysis suggests that demographics and labor market characteristics affect DI participation. Prior research has shown the importance of temporal changes in the age/sex composition of the labor force in explaining changes in DI participation (Daly, Lucking, and Schwabish 2013; Liebman 2015). In addition to these characteristics, decomposition analysis suggests that temporal changes in the population shares of people who are Hispanic, noncitizens, born outside of the United States, and speak a language other than English at home could also affect DI participation. Further research is needed to evaluate these trends. Prior research also indicates that changes in the industry composition of the labor market affect DI participation (Autor and Duggan 2003). This study reinforces those findings.
Lastly, the geographic analysis illustrates the wide between-state and within-state variation in socioeconomic conditions experienced by persons with disabilities. In some urban areas, conditions can vary substantially between geographic areas in close proximity. Because of this heterogeneity, the effects of DI/SSI reforms will likely vary across locations. The design of DI/SSI reforms, pilot programs, and evaluations will be strengthened by taking this heterogeneity into account.
Limitations
There are four limitations to this analysis. The first is possible bias. The decomposition estimates may be biased if an underreporting of disability prevalence is associated with DI/SSI participation. Our analysis suggests that if this bias exists, it is small and would not substantially change the findings.
The variance decomposition of DI/SSI participation is based on a simple mathematical relationship: The DI/SSI participation rate equals the disability prevalence rate multiplied by the DI/SSI participation rate among persons with disabilities. Thus, unlike the principal-components analysis and the regression-based decomposition of DI/SSI participation among persons with disabilities, the variance decomposition is a descriptive association rather than a causal one. Therefore, the second limitation is the descriptive rather than causal nature of the associations in the decomposition of DI/SSI participation among persons with disabilities.
Third, the DI participation rates used in this study include disabled workers but do not include disabled widow(er)s or disabled adult children because those data were not available in geographic detail. In 2011, there were approximately 8.5 million disabled-worker beneficiaries as well as 1.2 million disabled widow(er)s and disabled adult children (SSA 2015, Table 3). It is possible that the variance decomposition would change with the inclusion of disabled surviving spouses and disabled adult children. This limitation would be alleviated if PUMA-level counts of disabled widow(er)s and disabled adult children were publicly available.
Finally, the SSI participation rates used in this study include federal SSI and federally administered state supplementation recipients. The inclusion of the latter group introduces some interstate variation in SSI participation because federally administered state supplementation is available in some states and not others. The variance decomposition does not account for this variation. Because overall participation in federal SSI (6.5 million) is much higher than that for federally administered state supplementation (167,000; SSA 2012a, Table 7.A1), we do not expect this limitation to substantially affect our findings.
Conclusions
There is wide geographic variation in DI and SSI participation rates. Approximately 90 percent of the geographic variation can be attributed to geographic variation in disability prevalence, area socioeconomic characteristics, and the correlation between disability prevalence and DI/SSI participation among persons with disabilities.
Geographic variation in disability prevalence is a major reason for the wide variation in DI/SSI participation. If disability prevalence did not vary across areas, the geographic variation in DI participation would be reduced by approximately 80 percent and the variation in SSI participation would be reduced by approximately 63 percent. What accounts for the wide geographic variation in disability prevalence is not well understood. It may indicate cross-area disparities in public health, net migration of persons with and without disabilities, or other factors. Further research is needed to examine possible causes.
Notes
1 We exclude counties with fewer than 1,000 persons aged 18–64. See the Methods and Data sections for details on our sources and calculations.
2 We use the term “DI/SSI” to refer to participation in either program. Thus, it may include but is not restricted to concurrent participation in both programs.
3 For example, a 2011 Wall Street Journal article reported that “in 2010, 63% of [DI] applicants [in Puerto Rico] won approval, four percentage points higher than New Jersey and Wyoming, the most generous states” and that the program “is set to soon become the first big federal benefit program to run out of cash—and one of the main reasons is U.S. states and territories have a large say in who qualifies,” resulting in an “uneven selection process” (Paletta 2011).
4 Ben-Shalom and Stapleton (2014) estimate the ratios of DI and SSI participation to the working-age population with disabilities by state, but they do not analyze factors underlying the variances.
5 SSA requires that a person's disability has lasted or is expected to last for at least 1 year or to result in death and that a person is unable to engage in substantial gainful activity.
6 For additional details, see Gettens, Lei, and Henry (2016, 7–11).
7 See note 6.
8 If DI/SSI participants underreport their disability relative to nonparticipants, the biases will be the opposite of those described.
9 In our calculations, we impute the state mean SSI participation rates for those 104 counties.
10 In some instances, the smallest area of common PUMA and county boundaries contain multiple PUMAs and multiple counties.
11 Those four CAPUMAs include the following Kentucky counties: Rockcastle, Laurel, Jackson, Clay, Bell, Harlan, Knox, Whitley, Breathhitt, Knott, Lee, Leslie, Letcher, Owsley, Perry, Wolfe, Magoffin, Johnson, Floyd, Martin, and Pike.
12 We note that the names of the subcomponents are somewhat subjectively assigned. Brief and broadly descriptive terms, selected on the basis of the correlated variables, best suit our present purpose.
13 The association was determined by ordinary least squares regression estimates of equation 12.
14 Unobserved state policies are those that are not reflected in the variation of substate area characteristics. For example, substate variation in poverty, public assistance participation, and health insurance coverage rates may in part be due to variation in state policy.
15 For a description of the variables, data sources, detailed results, and estimates of the magnitude of the bias, see Gettens, Lei, and Henry (2016, 29–35). To further assess justification bias, we also compared the age profiles of disability prevalence and the age profiles of mortality, diabetes prevalence, and poor health. If justification bias were large, we would expect the increase in disability prevalence with age to exceed the increases with age in mortality, diabetes prevalence, or poor health because the increase in disability prevalence would reflect the justification bias associated with increased DI participation. We found that the age profiles are comparable, further suggesting that if justification bias exists, it is small.
16 We estimate the correlation between disability prevalence and program participation among persons with disabilities to be 0.13 for DI and 0.31 for SSI.
17 Furtado and Theodoropoulos (2012) found that social norms and information-sharing play important roles in SSI and DI participation among working-age immigrants.
References
Autor, David H., and Mark G. Duggan. 2003. “The Rise in Disability Rolls and the Decline in Unemployment.” Quarterly Journal of Economics 118(1): 157–206.
Ben-Shalom, Yonatan, and David Stapleton. 2014. National and State Program Participation Ratios for Working-Age People with Disabilities. Washington, DC: Mathematica Policy Research.
Black, Dan, Kermit Daniel, and Seth Sanders. 2002. “The Impact of Economic Conditions on Participation in Disability Programs: Evidence from the Coal Boom and Bust.” American Economic Review 92(1): 27–50.
Burkhauser, Richard V., J. S. Butler, and Robert R. Weathers II. 2001/2002. “How Policy Variables Influence the Timing of Applications for Social Security Disability Insurance.” Social Security Bulletin 64(1): 52–83.
Burkhauser, Richard V., Andrew J. Houtenville, and Jennifer R. Tennant. 2014. “Capturing the Elusive Working-Age Population with Disabilities: Reconciling Conflicting Social Success Estimates from the Current Population Survey and American Community Survey.” Journal of Disability Policy Studies, 24(4): 195–205.
Coe, Norma B., Kelly Haverstick, Alicia H. Munnell, and Anthony Webb. 2011. “What Explains State Variation in SSDI Application Rates?” CRR Working Paper No. 2011-23. Chestnut Hill, MA: Center for Retirement Research at Boston College. http://crr.bc.edu/working-papers/what-explains-state-variation-in-ssdi-application-rates/.
Daly, Mary C., Brian Lucking, and Jonathan A. Schwabish. 2013. “The Future of Social Security Disability Insurance.” FRBSF Economic Letter No. 2013-17. San Francisco, CA: Federal Reserve Bank of San Francisco. http://www.frbsf.org/economic-research/files/el2013-17.pdf.
Furtado, Delia, and Nikolaos Theodoropoulos. 2012. “Immigrant Networks and the Take-Up of Disability Programs: Evidence from U.S. Census Data.” CRR Working Paper No. 2012-23. Chestnut Hill, MA: Center for Retirement Research at Boston College.
Gettens, Jack, Pei-Pei Lei, and Alexis Henry. 2016. “Accounting for Geographic Variation in DI and SSI Participation.” DRC Working Paper 2016-03. Washington, DC: Mathematica Policy Research. https://www.mathematica-mpr.com/our-publications-and-findings/publications/accounting-for-geographic-variation-in-di-and-ssi-participation.
Guo, Xuguang (Steve), and John F. Burton, Jr. 2012. “The Growth in Applications for Social Security Disability Insurance: A Spillover Effect from Workers' Compensation.” Social Security Bulletin 72(3): 69–88.
Iyengar, Radha, and Giovanni Mastrobuoni. 2014. “The Political Economy of the Disability Insurance: Theory and Evidence of Gubernatorial Learning.” IZA Journal of Labor Policy 3(1): 1–31.
Keiser, Lael R. 2001. “Street-Level Bureaucrats, Administrative Power and the Manipulation of Federal Social Security Disability Programs.” State Politics and Policy Quarterly 1(2): 144–164.
Liebman, Jeffrey B. 2015. “Understanding the Increase in Disability Insurance Benefit Receipt in the United States.” Journal of Economic Perspectives 29(2): 123–150.
Maestas, Nicole, Kathleen J. Mullen, and Alexander Strand. 2013. “Disability Insurance and Healthcare Reform: Evidence from Massachusetts.” MRRC Working Paper No. WP 2013-289. Ann Arbor, MI: University of Michigan Retirement Research Center. http://www.mrrc.isr.umich.edu/publications/papers/pdf/wp289.pdf.
McCoy, John L., Miles Davis, and Russell E. Hudson. 1994. “Geographic Patterns of Disability in the United States.” Social Security Bulletin 57(1): 25–36.
McVicar, Duncan. 2006. “Why Do Disability Benefit Rolls Vary Between Regions? A Review of the Evidence from the USA and the UK.” Regional Studies 40(5): 519–533.
Nelson, William J., Jr. 1994. “Disability Trends in the United States: A National and Regional Perspective.” Social Security Bulletin 57(3): 27–41.
Paletta, Damian. 2011. “Insolvency Looms as States Drain U.S. Disability Fund.” Wall Street Journal, March 22.
Pattison, David, and Hilary Waldron. 2013. “Growth in New Disabled-Worker Entitlements, 1970–2008.” Social Security Bulletin 73(4): 25–48.
Ruffing, Kathy A. 2015. “Geographic Pattern of Disability Receipt Largely Reflects Economic and Demographic Factors.” Washington, DC: Center on Budget and Policy Priorities. https://www.cbpp.org/sites/default/files/atoms/files/1-8-15ss.pdf.
Rupp, Kalman. 2012. “Factors Affecting Initial Disability Allowance Rates for the Disability Insurance and Supplemental Security Income Programs: The Role of the Demographic and Diagnostic Composition of Applicants and Local Labor Market Conditions.” Social Security Bulletin 72(4): 11–35.
Rupp, Kalman, and David Stapleton. 1995. “Determinants of the Growth in the Social Security Administration's Disability Programs—An Overview.” Social Security Bulletin 58(4): 43–70.
Rupp, Kalman, and David C. Stapleton, editors. 1998. Growth in Disability Benefits: Explanations and Policy Implications. Kalamazoo, MI: W. E. Upjohn Institute for Employment Research.
Rutledge, Matthew S. 2012. “The Impact of Unemployment Insurance Extensions on Disability Insurance Application and Allowance Rates.” CRR Working Paper No. 2011-17. Chestnut Hill, MA: Center for Retirement Research at Boston College.
Rutledge, Matthew S., and April Yanyuan Wu. 2014. “Why Do SSI and SNAP Enrollments Rise in Good Economic Times and Bad?” CRR Working Paper No. 2014-10. Chestnut Hill, MA: Center for Retirement Research at Boston College.
Social Security Administration. 2012a. Annual Statistical Supplement to the Social Security Bulletin, 2011. Washington, DC: SSA. https://www.ssa.gov/policy/docs/statcomps/supplement/2011/index.html.
———. 2012b. OASDI Beneficiaries by State and County, 2011. Washington, DC: SSA. https://www.ssa.gov/policy/docs/statcomps/oasdi_sc/2011/index.html.
———. 2012c. SSI Recipients by State and County, 2011. Washington, DC: SSA. https://www.ssa.gov/policy/docs/statcomps/ssi_sc/2011/index.html.
———. 2015. Annual Statistical Report on the Social Security Disability Insurance Program, 2014. Washington, DC: SSA. https://www.ssa.gov/policy/docs/statcomps/di_asr/2014/index.html.
Social Security Advisory Board. 2001. Charting the Future of Social Security's Disability Programs: The Need for Fundamental Change. Washington, DC: Social Security Advisory Board.
———. 2012a. Aspects of Disability Decision Making: Data and Materials. Washington, DC: Social Security Advisory Board.
———. 2012b. Filing for Social Security Disability Benefits: What Impact Does Professional Representation Have on the Process at the Initial Application Level? Washington, DC: Social Security Advisory Board.
Soss, Joe, and Lael R. Keiser. 2006. “The Political Roots of Disability Claims: How State Environments and Policies Shape Citizen Demands.” Political Research Quarterly 59(1): 133–148.
[SSA] See Social Security Administration.
Strand, Alexander. 2002. “Social Security Disability Programs: Assessing the Variation in Allowance Rates.” ORES Working Paper Series No. 98. Washington, DC: SSA.
Woehl, Erica M. 2015 “Social Security Administration Disability Discrepancies: The Debate between Street-Level Bureaucrats and Administrative Law Judges.” North Dakota Law Review 91: 353–373.
Zayatz, Tim. 2015. “Social Security Disability Insurance Program Worker Experience.” Actuarial Study No. 123. Baltimore, MD: SSA, Office of the Chief Actuary.